DePIN 浏览器 - DePIN Scan
趋势 🔥
DePIN 市值
$27,927,169,073
-0.6%
交易量
$11,276,084,035
-1.4%
DePIN项目
296
DePIN设备
19,074,201
+0.1%
DePIN 项目
DePIN Scan是DePIN加密项目的浏览器。有296个DePIN项目,总共的DePIN市值为$27,927,169,073,总共的DePIN设备数量为19,074,201。点击下面的项目了解如何从今天开始赚取被动收入。
项目 | 代币 | 类别 | 社交关注 | 市值 | 代币价格 | 24小时交易量 | 1天 | 7天 | 30天 | 设备总数 | 最近7天 |
---|---|---|---|---|---|---|---|---|---|---|---|
SOL | Chain | 2,857,235 | $120,782,319,764 | $254.55 | $9,591,230,620 | -0.4% | +20.5% | +49.0% | - | ||
![]() | RNDR | ServerAI | 205,471 | $3,751,325,656 | $7.25 | $339,115,875 | -2.3% | +9.1% | +46.1% | - | |
![]() | FIL | Server | 664,476 | $2,866,147,653 | $4.77 | $490,907,794 | +5.1% | +13.6% | +30.8% | 3,539 | |
![]() | THETA | ServerAI | 270,337 | $1,690,944,887 | $1.69 | $73,542,809 | -1.8% | +24.3% | +36.4% | 5,885 | |
![]() | AKT | ServerAI | 119,054 | $921,029,389 | $3.72 | $29,051,408 | -1.6% | +18.5% | +53.5% | 472 | |
![]() | HNT | Wireless | 213,792 | $914,878,176 | $5.32 | $20,007,525 | -6.6% | -8.8% | -23.5% | - | |
![]() | GRASS | ComputeAI | 514,215 | $515,596,844 | $2.11 | $214,964,877 | -14.2% | -23.6% | - | - | |
IOTX | Chain | 300,755 | $390,110,892 | $0.04134 | $23,866,820 | -2.0% | +7.9% | +7.0% | - | ||
![]() | IO | ComputeAI | 506,482 | $291,328,998 | $2.42 | $175,337,925 | +10.6% | +18.5% | +38.2% | - | |
![]() | NOS | ComputeAI | 59,263 | $265,477,857 | $3.18 | $3,532,329 | -11.4% | -1.3% | +50.0% | - | |
![]() | ATH | Compute | 844,097 | $233,494,707 | $0.05751 | $44,708,871 | -3.8% | -4.7% | +16.6% | - | |
![]() | HONEY | SensorAI | 48,864 | $186,376,849 | $0.06348 | $1,032,126 | +1.1% | -9.7% | +21.8% | 8,037 |
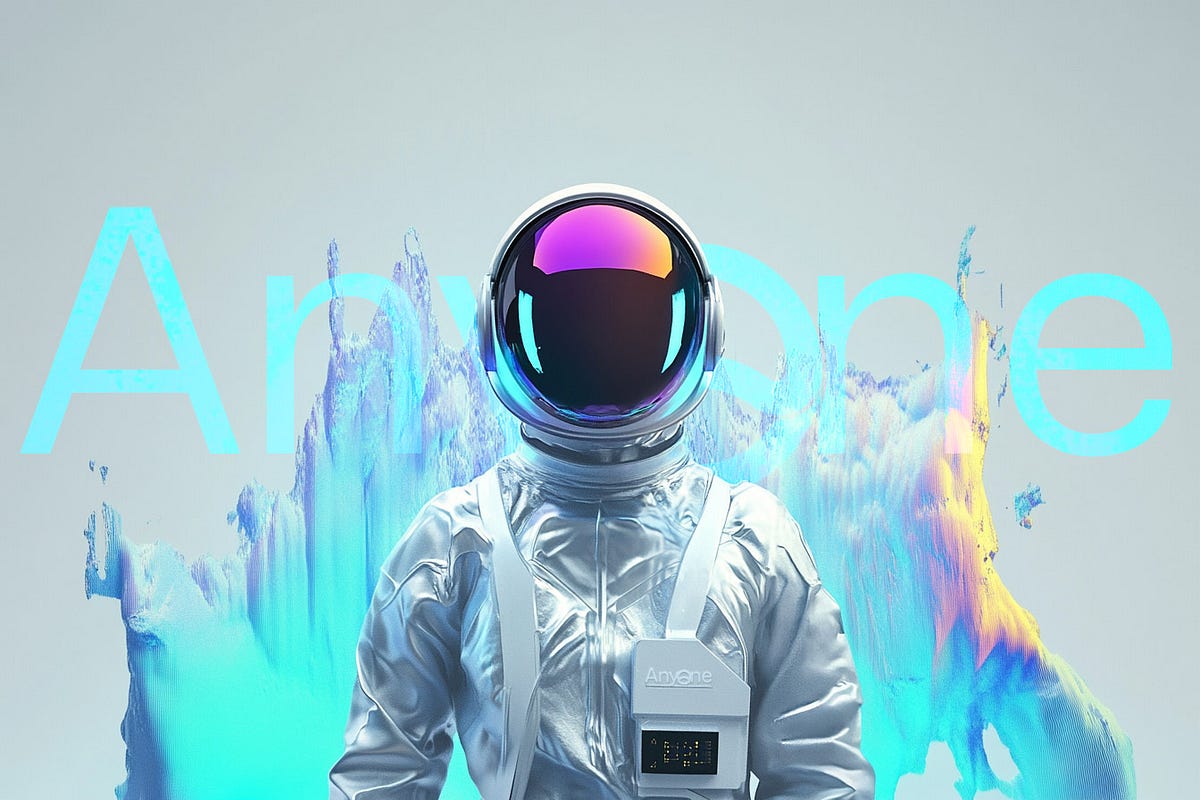
21 小时前
ATOR的旅程:构建去中心化的互联网隐私未来一年前,Tor项目决定从其网络中移除所有ATOR中继,开启了去中心化未来的愿景。团队致力于创建一个自我扩展、无需信任的网络,以增强全球用户的隐私,目标是通过定制硬件颠覆VPN市场,使私人浏览服务惠及数十亿人。现已推出的Anyone Network拥有5000个活跃中继,成为全球最大、最快的混合网络之一。团队感谢社区在艰难时期的支持,展现了去中心化和社区协作的力量。项目已转型为开源倡议,从单一的Smartweave注册协议扩展到30多个活跃代码库,涵盖区块链技术、隐私、硬件和应用。展望未来,团队计划在过渡到AO分发协议后进一步去中心化,并强调社区治理在网络安全和性能中的重要性。ATOR的精神象征着在Web3领域克服挫折的决心,团队承诺通过定期更新保持透明,致力于推动全球隐私的普及。
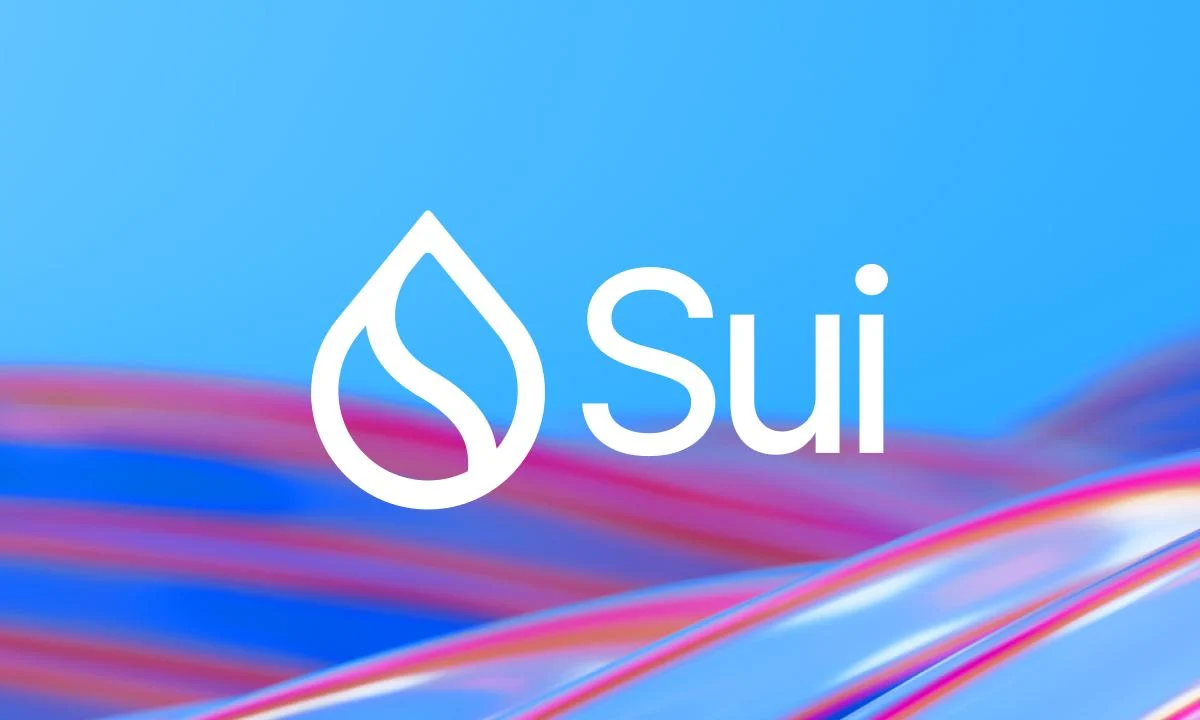
21 小时前
Sui基金会与富兰克林坦普顿合作推动区块链创新2024年11月22日,Sui基金会宣布与富兰克林坦普顿数字资产达成战略合作,旨在推动Sui区块链生态系统的创新。此次合作将促进Sui这一Layer 1智能合约平台的发展,为区块链解决方案的建设者创造价值机会。Sui生态系统开发负责人Jameel Khalfan表示,此次合作验证了Sui技术的有效性,富兰克林坦普顿正在解决去中心化金融中的挑战。富兰克林坦普顿数字资产在区块链技术方面已有多年经验,专注于构建解决方案和投资策略。Sui生态系统内已有多个创新项目,如去中心化金融解决方案DeepBook和支持去中心化移动网络的Karrier One。此外,Sui最近推出了原生USDC,为用户提供直接访问广泛使用的稳定币的机会,进一步提升了其作为安全可扩展平台的地位,吸引各行业的应用开发者。
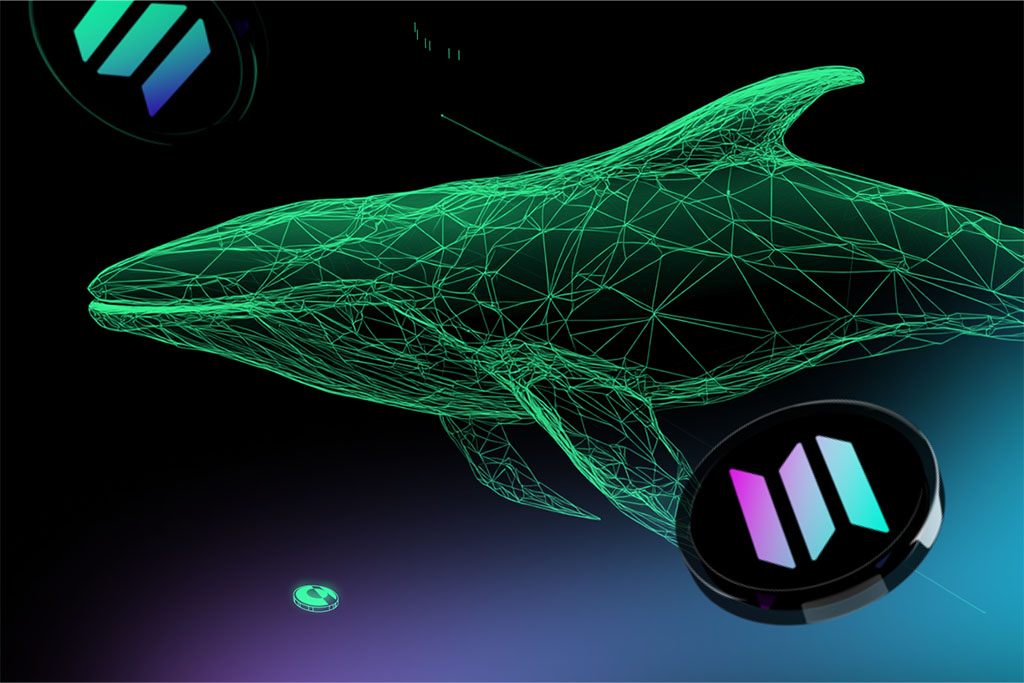
21 小时前
Akash网络与Lunex网络引领加密货币创新加密货币市场正在经历重要发展,Akash网络的DePIN协议市值已突破十亿美元,推动Akash价格上涨,反映出投资者信心增强。同时,Solana即将创下新高,市值流入使其超越BNB,预示着主流采用和强劲发展潜力。在去中心化金融(DeFi)领域,Lunex网络作为新兴玩家,提供零手续费的跨链交易,拥有超过50,000个交易对,旨在简化DeFi,吸引零售投资者。Lunex网络已获得295万美元的流动性,力图成为DeFi领域的关键参与者。Akash网络、Solana和Lunex网络的崛起,显示出去中心化创新的趋势,Akash在过去24小时内上涨10%,一周内增长36%,展现出颠覆传统云服务的潜力。投资者正密切关注这些动态。
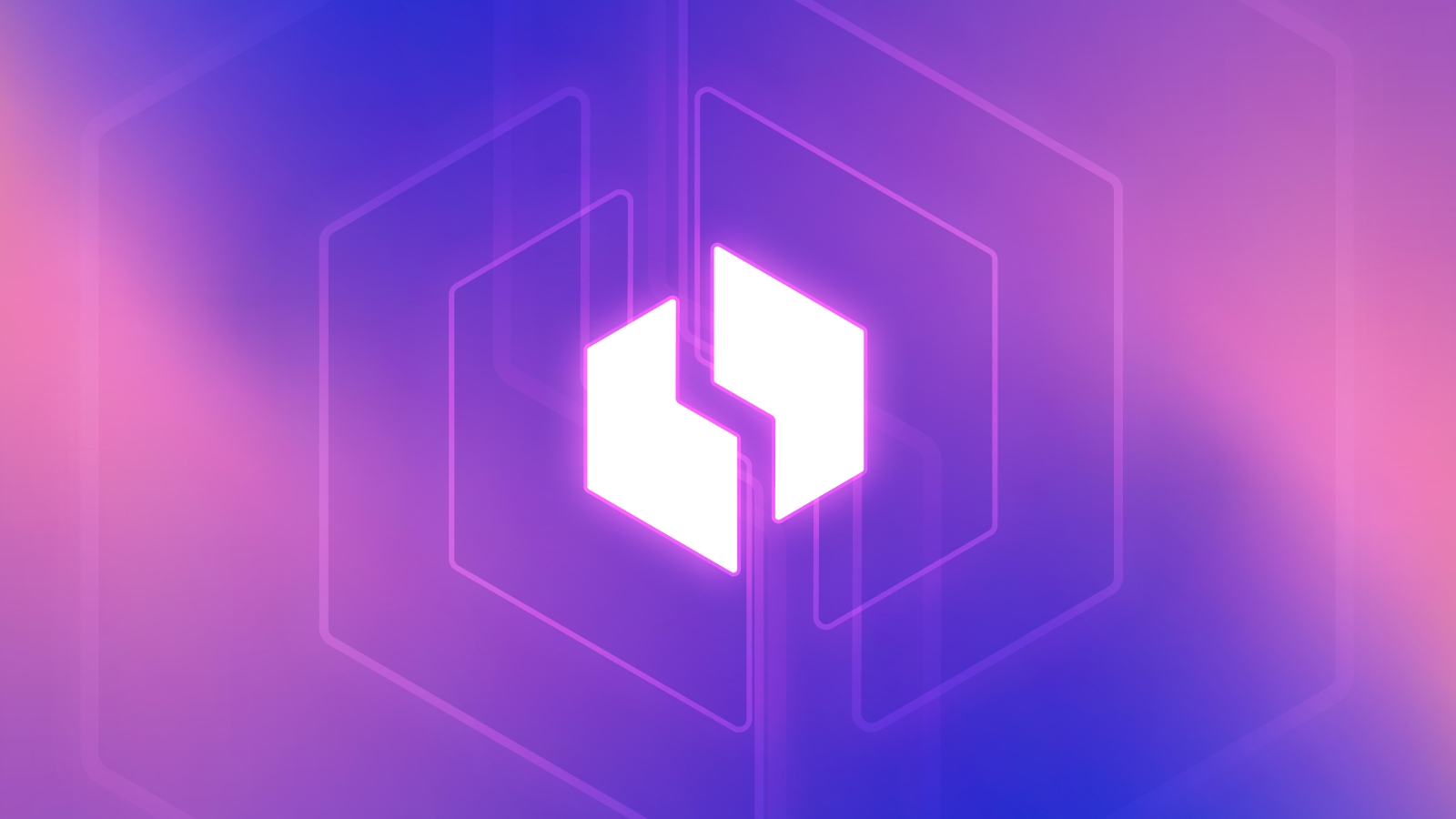
21 小时前
Enhancing Context Recall in Retrieval-Augmented GenerationRetrieval-augmented generation (RAG) has emerged as a pivotal method for integrating large language models (LLMs) into specialized business applications, enabling the infusion of proprietary data into model responses. Despite its effectiveness during the proof of concept (POC) phase, developers often face significant accuracy drops when transitioning RAG into production. This issue is particularly pronounced during the retrieval phase, where the aim is to accurately fetch the most relevant context for a given query, a metric known as context recall. This article delves into strategies for enhancing context recall by customizing and fine-tuning embedding models, ultimately improving RAG's performance in real-world applications.
RAG operates in two main steps: retrieval and generation. In the retrieval phase, the model converts text into vectors, indexes, retrieves, and re-ranks these vectors to identify the top matches. However, failures in this phase can lead to missed relevant contexts, resulting in lower context recall and less accurate generation outputs. One effective solution is to adapt the embedding model, which is designed to understand relationships between text data, to produce embeddings that are specific to the dataset being used. This fine-tuning allows the model to generate similar vectors for similar sentences, enhancing its ability to retrieve context that is highly relevant to the query.
To improve context recall, it is essential to prepare a tailored dataset that reflects the types of queries the model will encounter. This involves extracting a diverse range of questions from the knowledge base, paraphrasing them for variability, and organizing them by relevance. Additionally, constructing an evaluation dataset helps assess the model's performance in a realistic setting. By employing an Information Retrieval Evaluator, developers can measure metrics like Recall@k and Precision@k to gauge retrieval accuracy. Ultimately, fine-tuning the embedding model can lead to substantial improvements in context recall, ensuring that RAG remains accurate and reliable in production environments.