Fine-Tuning Llama 3.2 11B with Q-LoRA for Extractive Question Answering
Large Language Models (LLMs) have become essential tools in natural language processing, capable of handling a variety of tasks. However, due to their broad training, they may not excel in specific applications without further adaptation. Fine-tuning techniques, such as Q-LoRA, allow researchers to tailor pre-trained models like Llama 3.2 11B for particular tasks, such as extractive question answering. This article outlines the process of fine-tuning Llama 3.2 11B using Q-LoRA on the SQuAD v2 dataset, showcasing the performance enhancements achieved through this method.
LoRA, or Low-Rank Adaptation, is a technique that introduces new weights to an existing model without altering the original parameters. By adding adapter weights that adjust the outputs of certain layers, LoRA enables models to retain their pre-trained knowledge while acquiring new capabilities tailored to specific tasks. In this experiment, the focus is on fine-tuning Llama 3.2 11B for extractive question answering, aiming to extract precise text segments that answer user queries directly, rather than summarizing or rephrasing the content. The experiment was conducted on a Google Colab platform utilizing an A100 GPU, with the Hugging Face Transformers library facilitating the implementation.
The results of the fine-tuning process were promising, demonstrating a significant boost in the model’s performance on the validation set. The BERT score improved from 0.6469 to 0.7505, while the exact match score rose from 0.116 to 0.418. These enhancements indicate that the Q-LoRA technique effectively adapts the Llama 3.2 11B model for extractive question answering tasks. This article serves as a guide for researchers looking to apply similar methods to other models and tasks, highlighting the potential of fine-tuning in the realm of natural language processing.
Related News
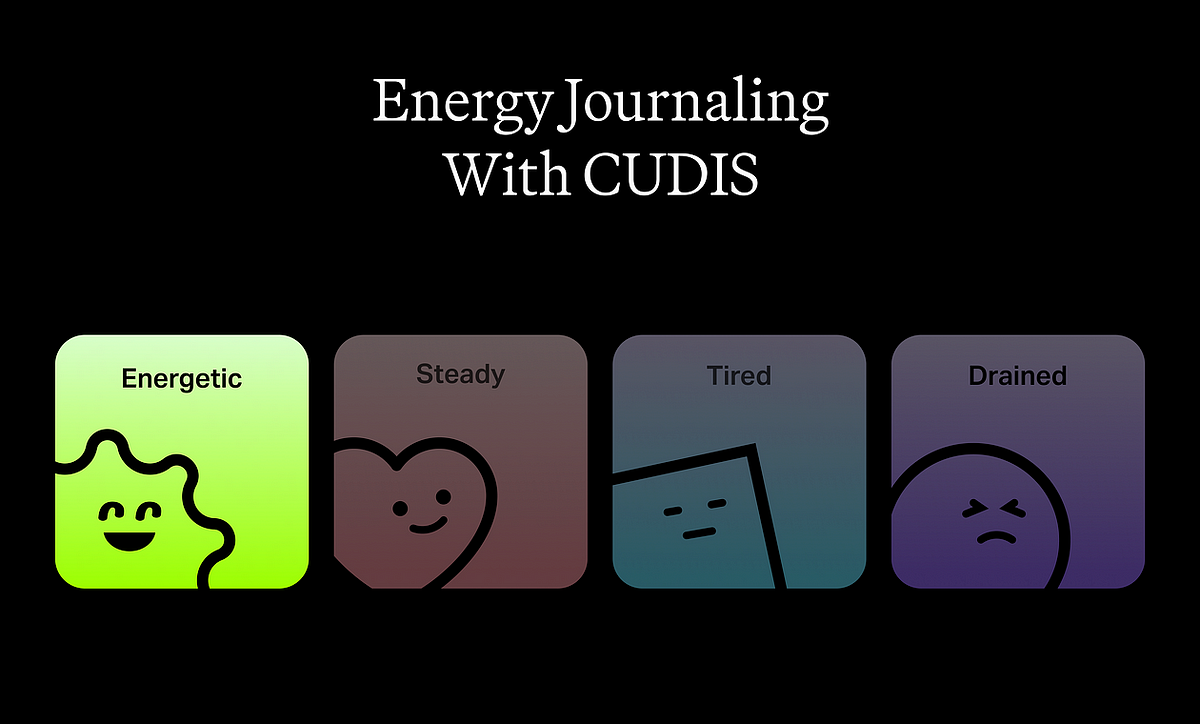
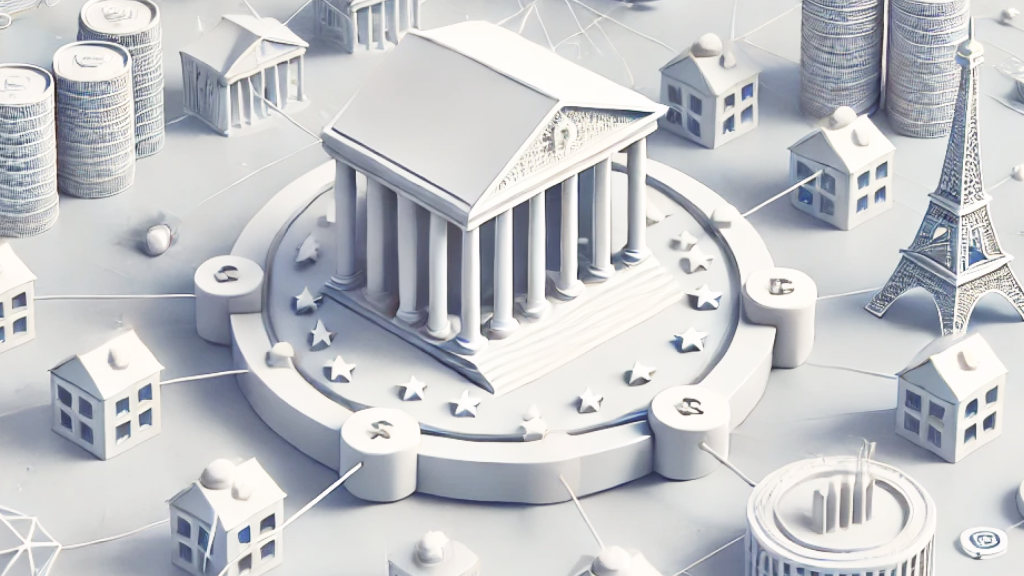
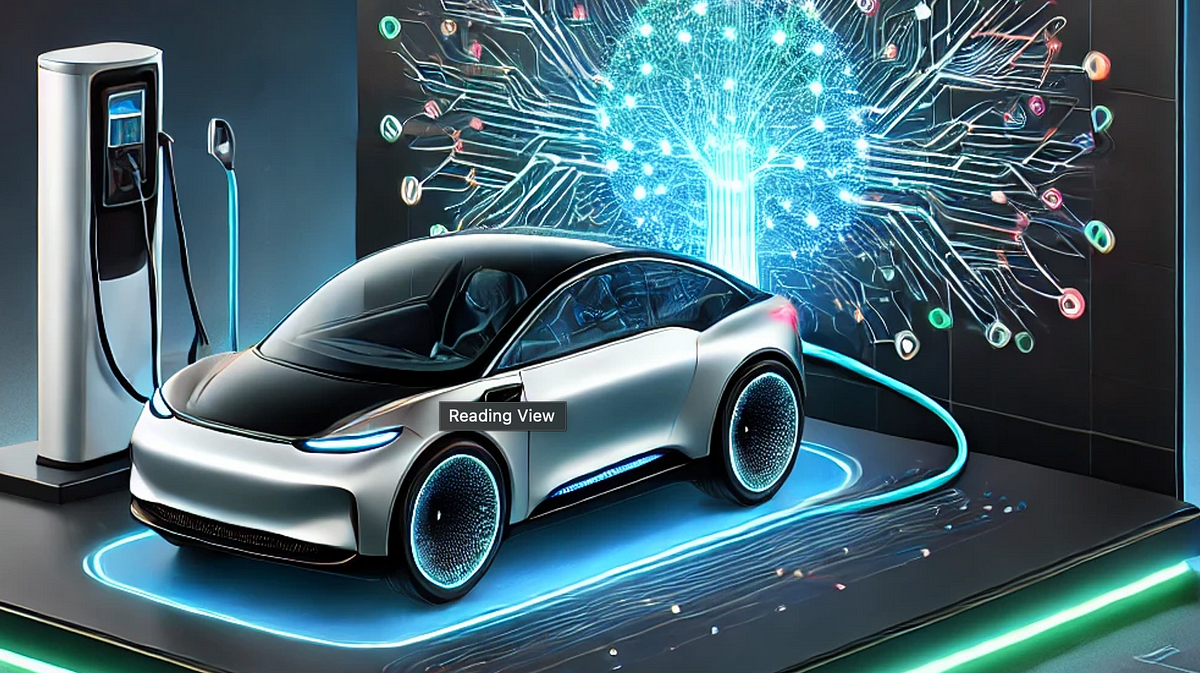
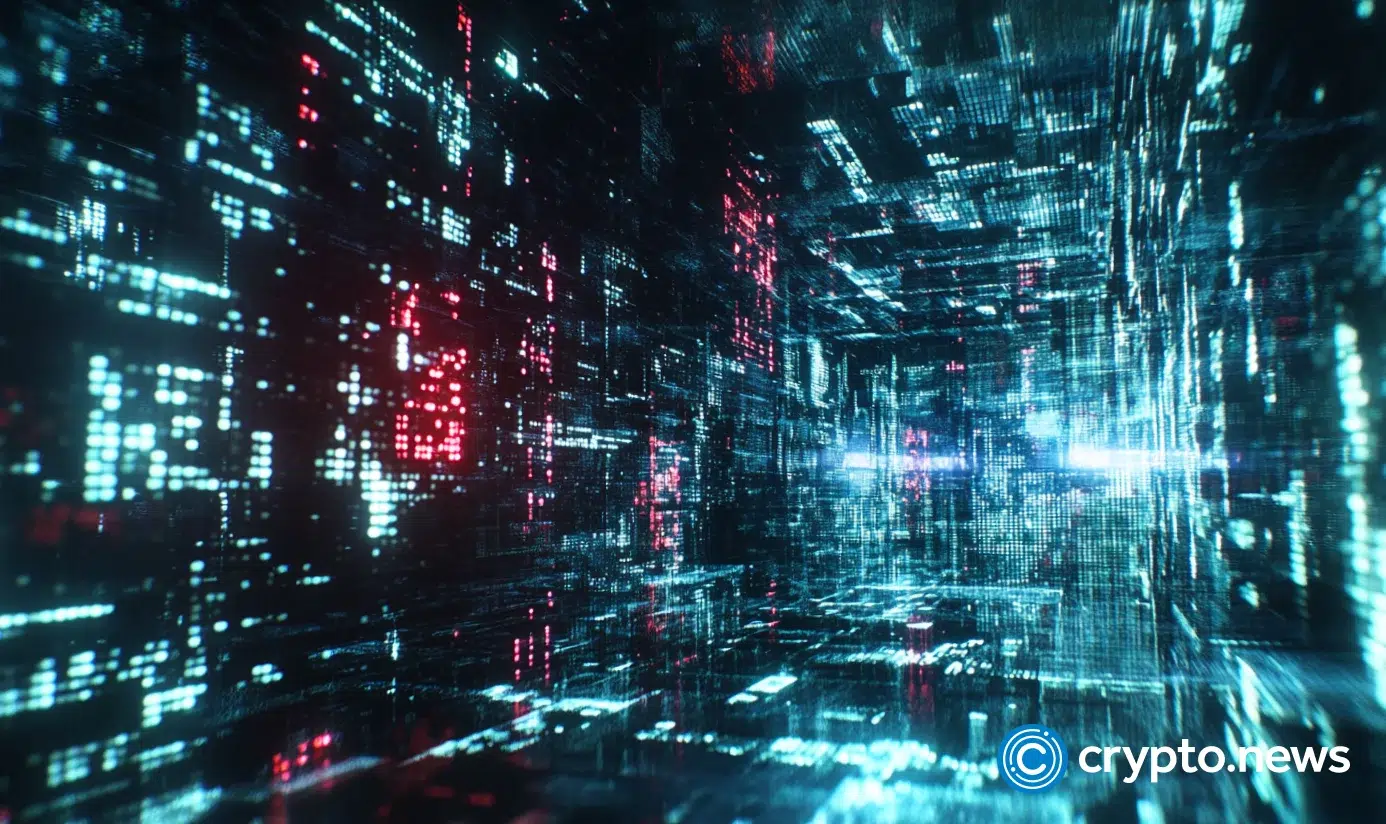

