Revolutionizing AI Efficiency: The Impact of the L-Mul Algorithm
The rapid development of artificial intelligence (AI) has led to significant advancements across various sectors, yet it comes with a hefty environmental price tag due to its high energy consumption. AI models, particularly those utilizing neural networks, require substantial computational power, which translates to enormous electricity usage. For example, running ChatGPT in early 2023 consumed approximately 564 MWh of electricity daily, equivalent to the energy needs of around 18,000 U.S. households. This energy demand is primarily driven by complex floating-point operations essential for neural network computations, making the search for energy-efficient solutions critical as AI systems grow in complexity.
Enter the L-Mul (Linear-Complexity Multiplication) algorithm, a groundbreaking development that promises to significantly reduce the energy burden associated with AI computations. L-Mul operates by approximating floating-point multiplications with simpler integer additions, which can be integrated into existing AI models without the need for fine-tuning. This innovative approach has demonstrated remarkable energy savings, achieving up to 95% reduction in energy consumption for element-wise tensor multiplications and 80% for dot product computations. Importantly, this energy efficiency does not compromise the accuracy of AI models, marking a significant advancement in the quest for sustainable AI.
The implications of L-Mul extend beyond mere energy savings; it enhances the performance of AI models across various applications, including transformer models and large language models (LLMs). In benchmarks such as GSM8k and visual question answering tasks, L-Mul has outperformed traditional floating-point formats like FP8, showcasing its potential to handle complex computations efficiently. As the demand for AI continues to rise, L-Mul stands out as a pivotal solution that not only addresses the energy crisis associated with AI but also paves the way for a more sustainable future in technology development.
Related News
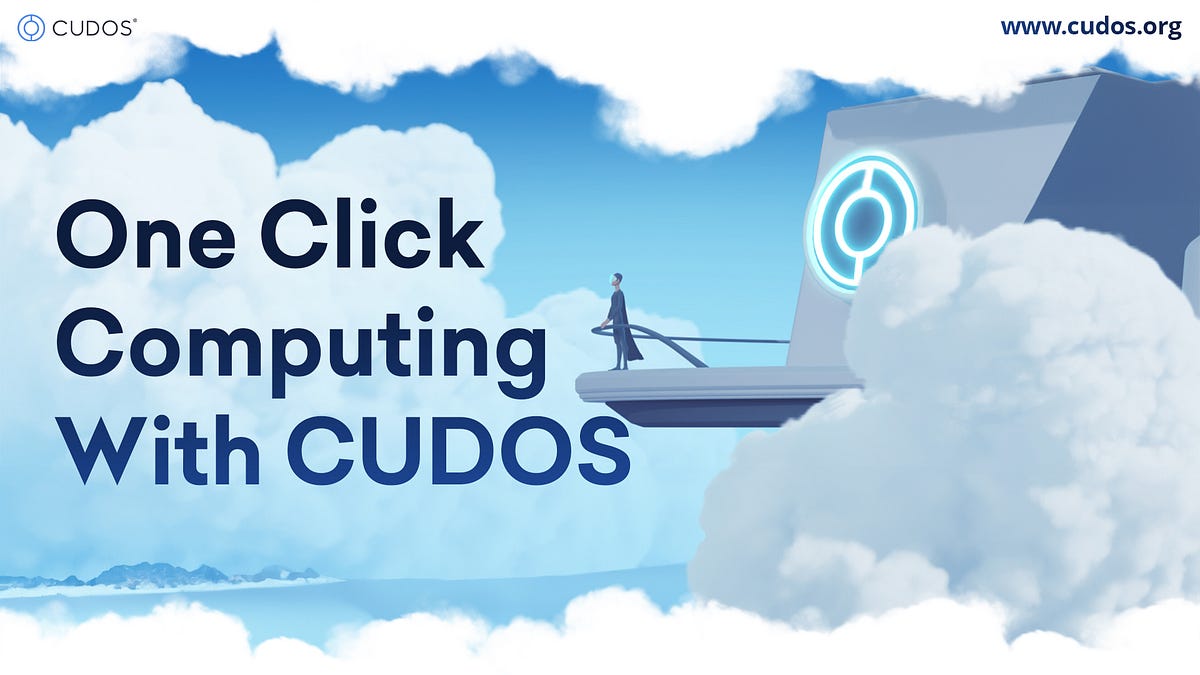
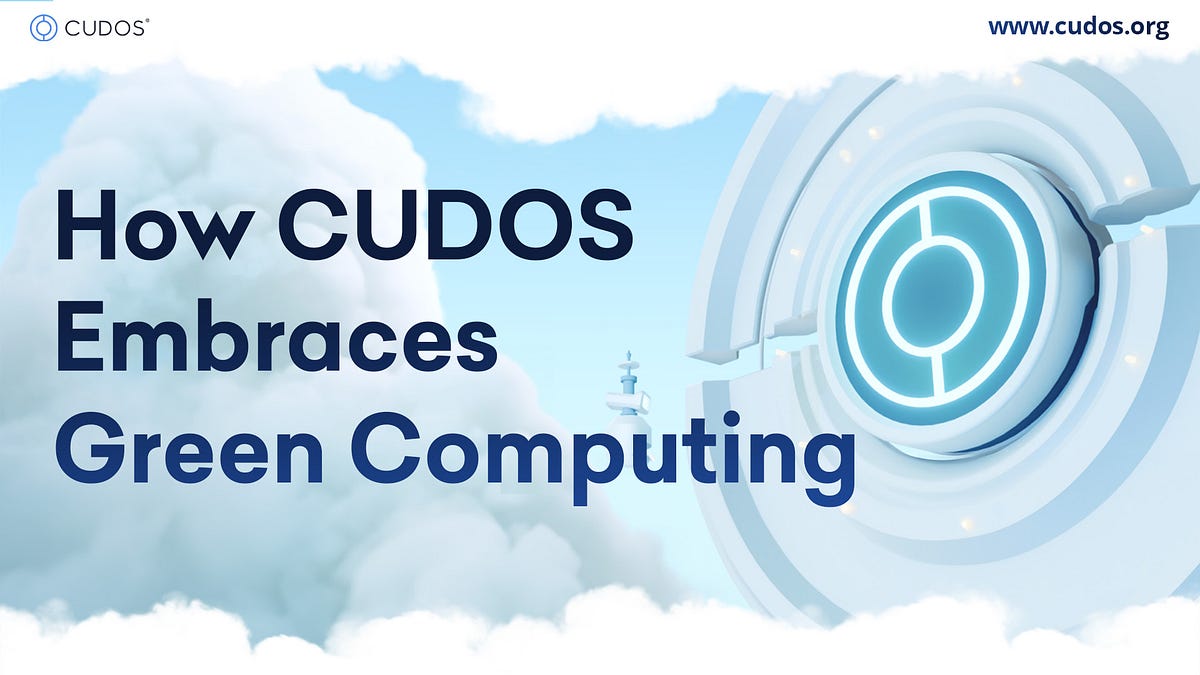
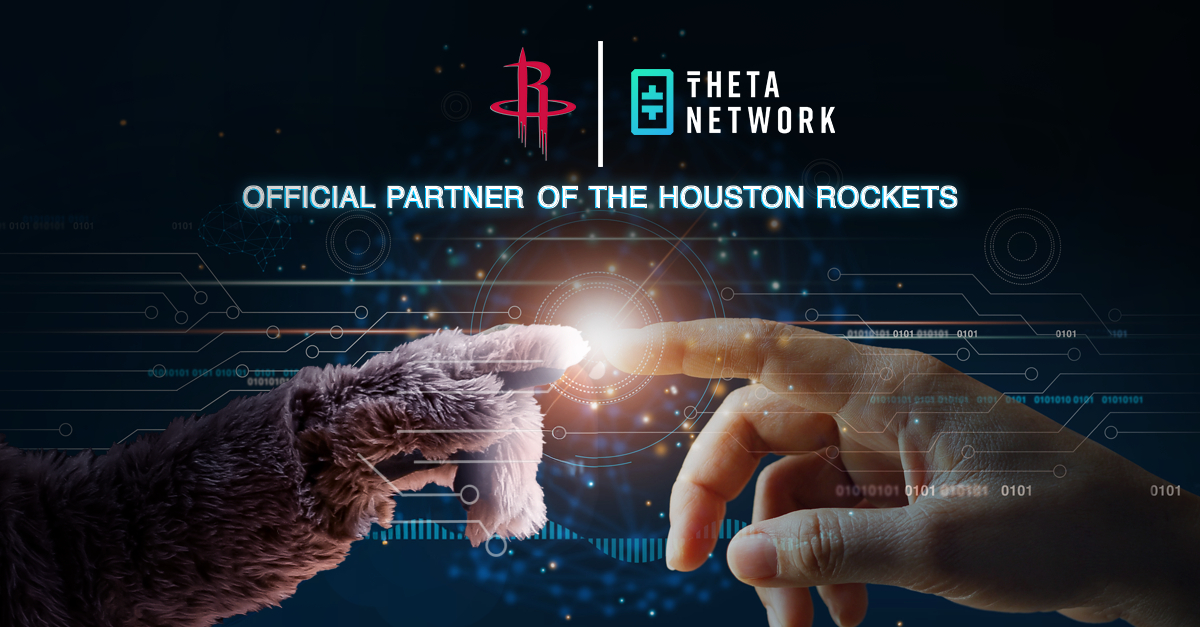
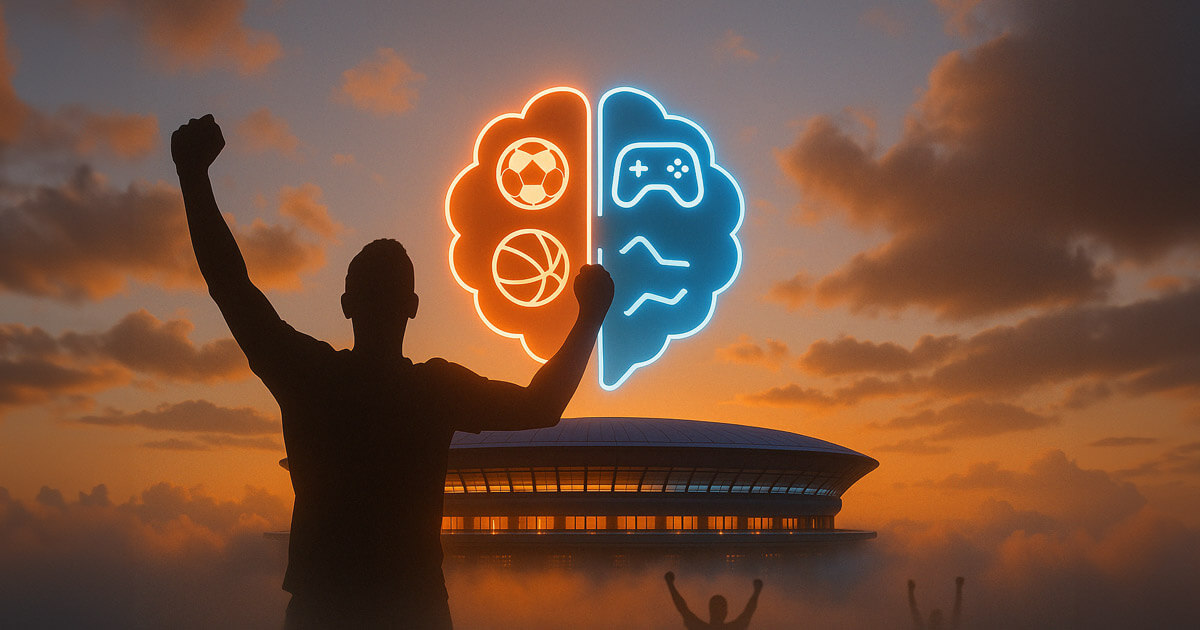

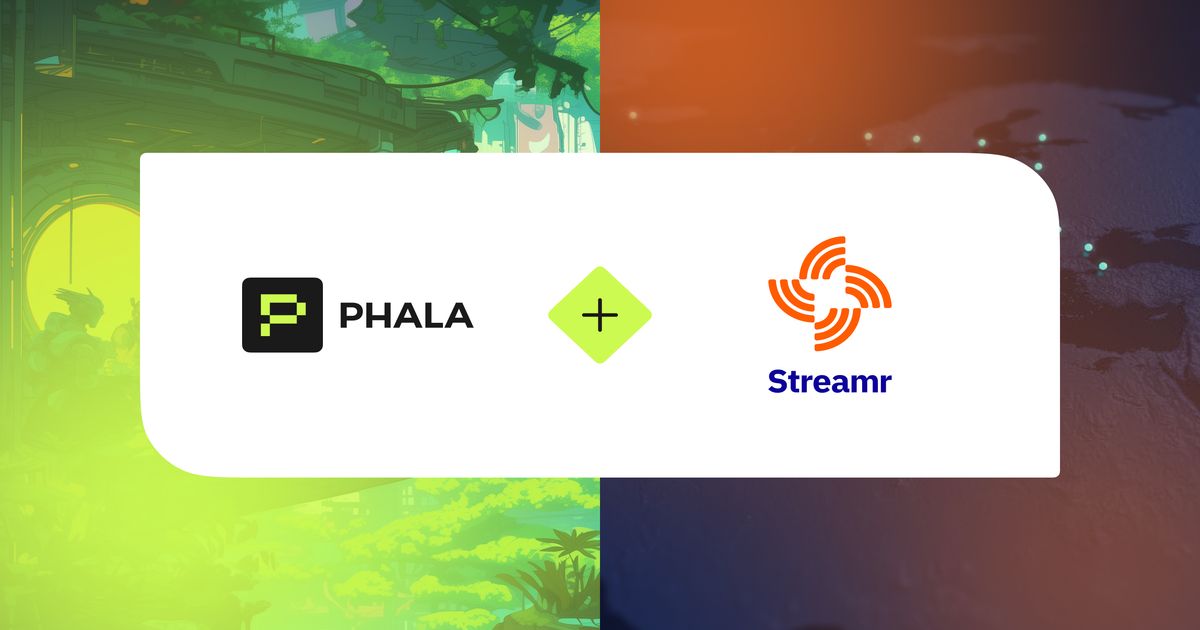