Falcon Mamba 7B: A Breakthrough in Attention-Free AI Models
The rapid evolution of artificial intelligence (AI) is significantly influenced by the emergence of attention-free models, with Falcon Mamba 7B being a notable example. Developed by the Technology Innovation Institute (TII) in Abu Dhabi, this groundbreaking model departs from traditional Transformer-based architectures that rely heavily on attention mechanisms. Instead, Falcon Mamba 7B utilizes State-Space Models (SSMs), which provide faster and more memory-efficient inference, addressing the computational challenges associated with long-context tasks. By training on an extensive dataset of 5.5 trillion tokens, Falcon Mamba 7B positions itself as a competitive alternative to existing models like Google’s Gemma and Microsoft’s Phi.
Falcon Mamba 7B’s architecture is designed to maintain a constant inference cost, regardless of input length, effectively solving the quadratic scaling problem that plagues Transformer models. This unique capability allows it to excel in applications requiring long-context processing, such as document summarization and customer service automation. While it has demonstrated superior performance in various natural language processing benchmarks, it still faces limitations in tasks that demand intricate contextual understanding. Nevertheless, its memory efficiency and speed make it a compelling choice for organizations looking to optimize their AI solutions.
The implications of Falcon Mamba 7B extend beyond mere performance metrics. Its support for quantization enables efficient deployment on both GPUs and CPUs, further enhancing its versatility. As the AI landscape evolves, the success of Falcon Mamba 7B suggests that attention-free models may soon become the standard for many applications. With ongoing research and development, these models could potentially surpass traditional architectures in both speed and accuracy, paving the way for innovative applications across various industries.
Related News
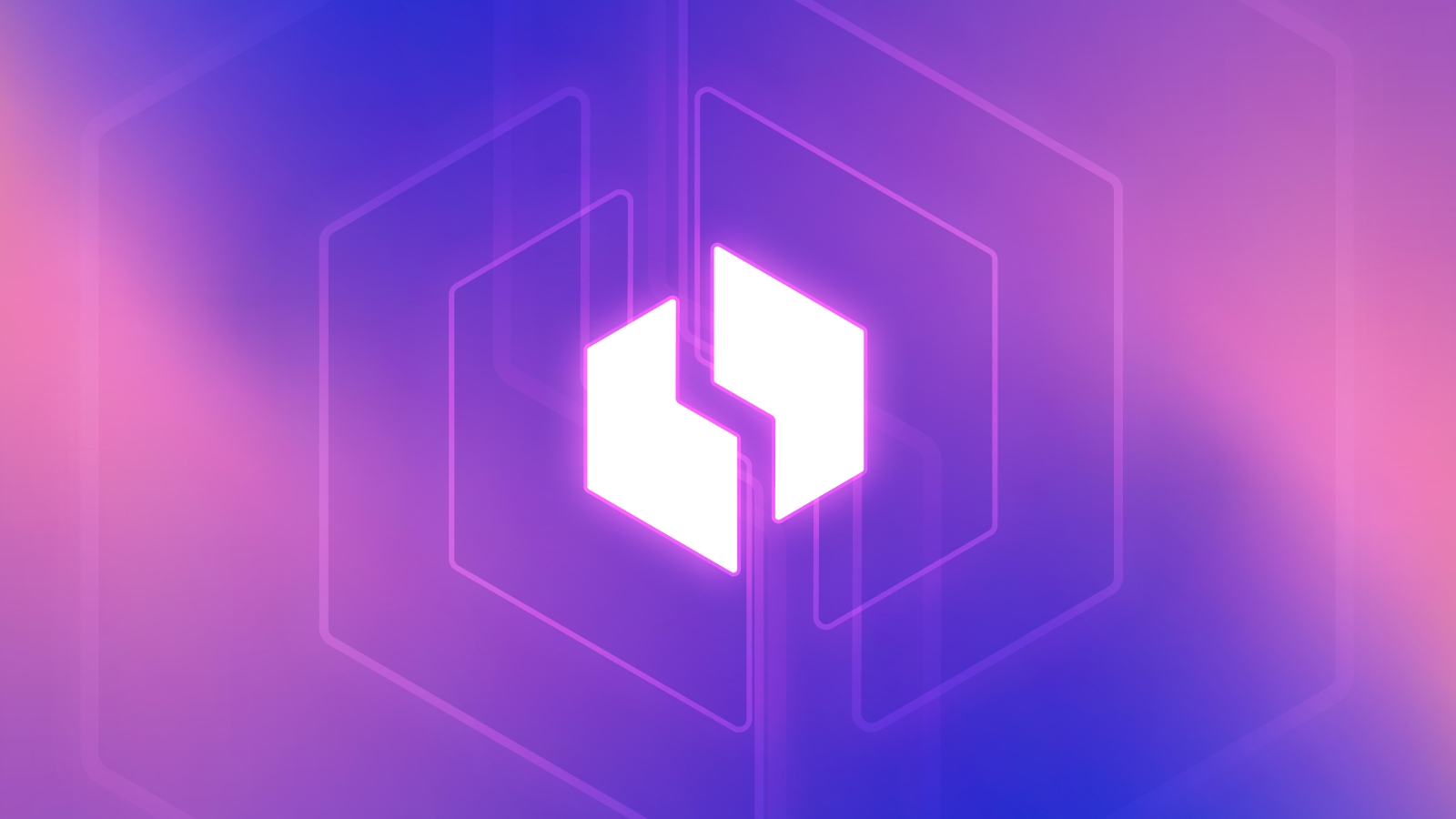
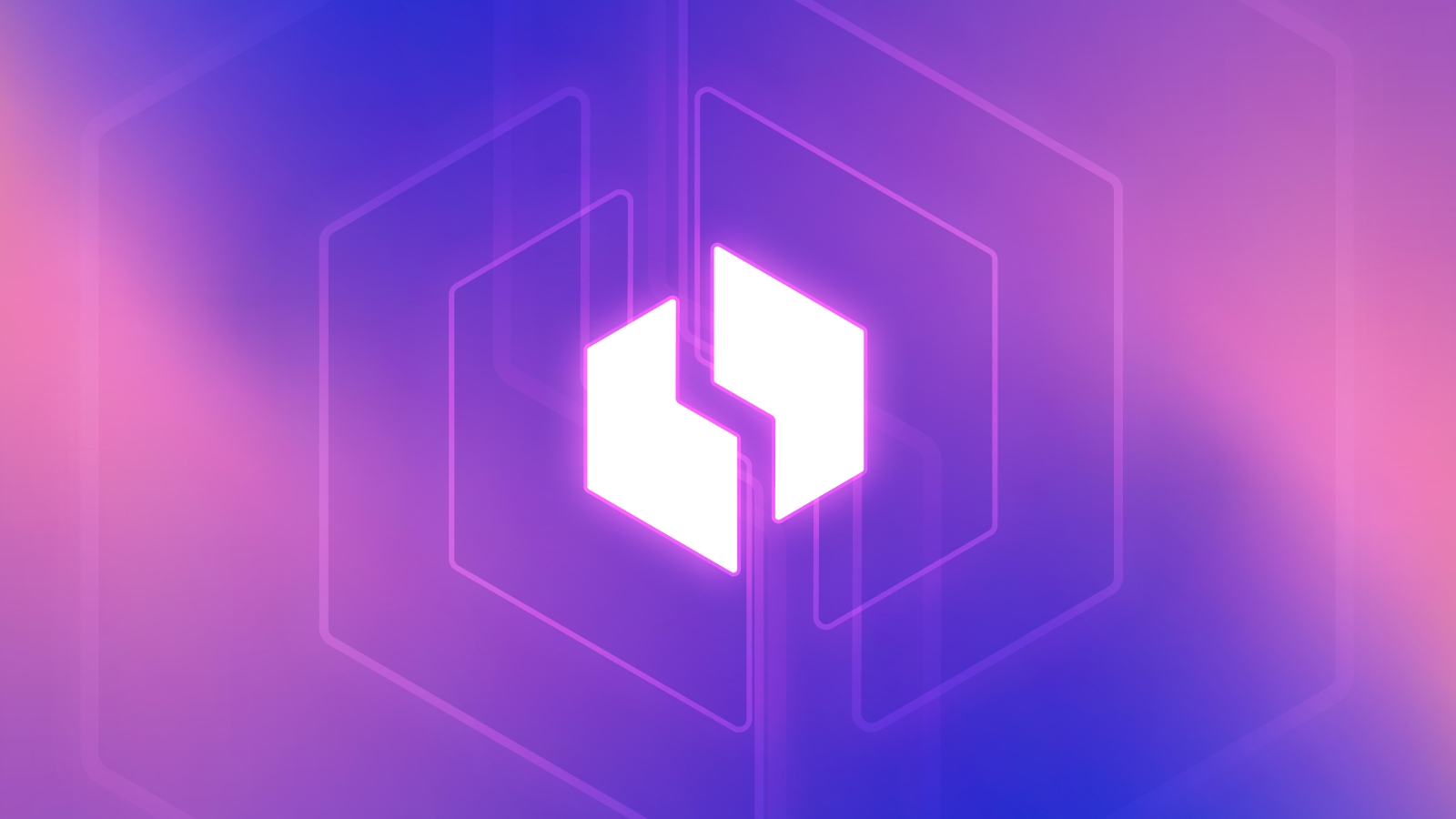
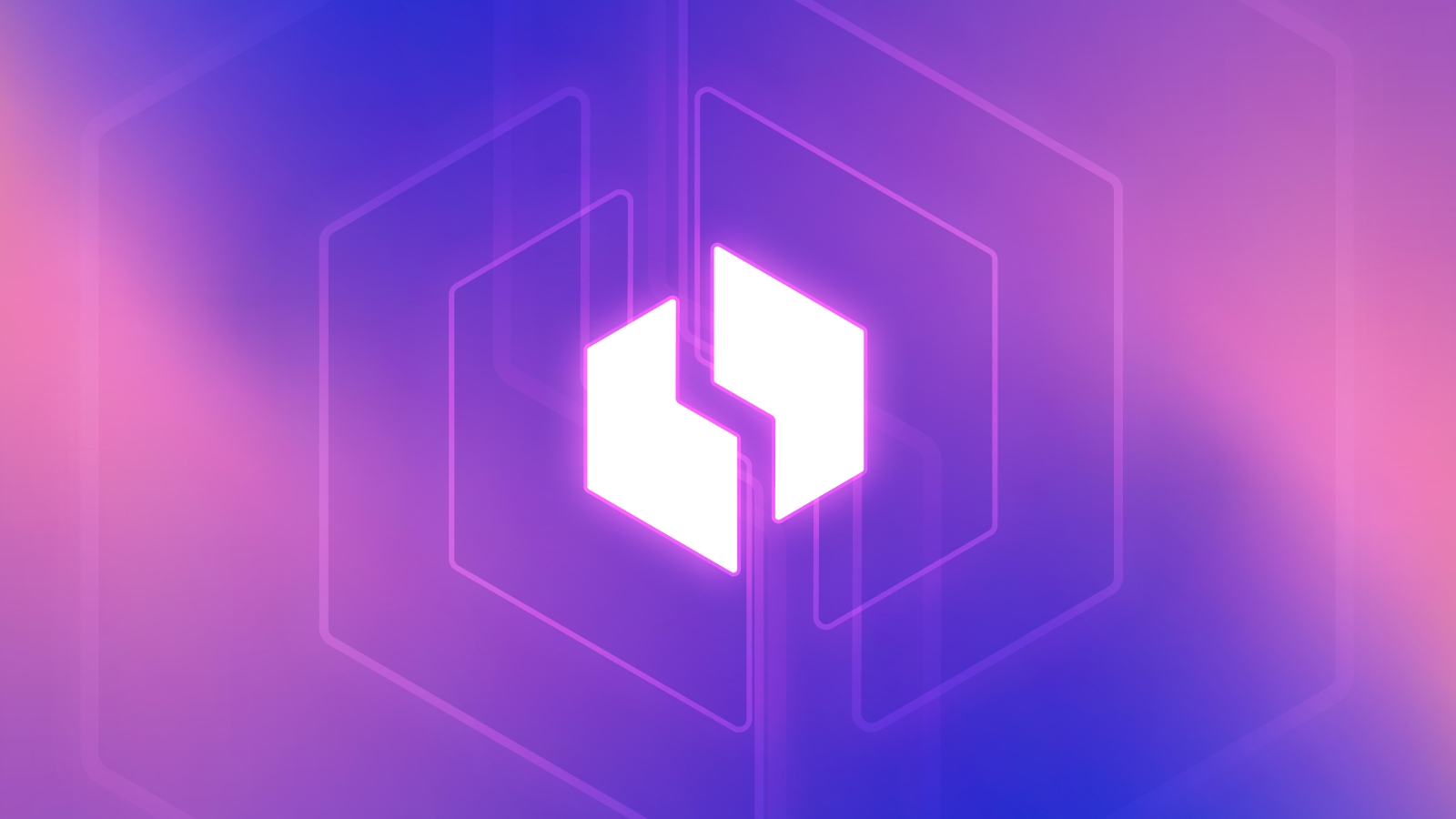
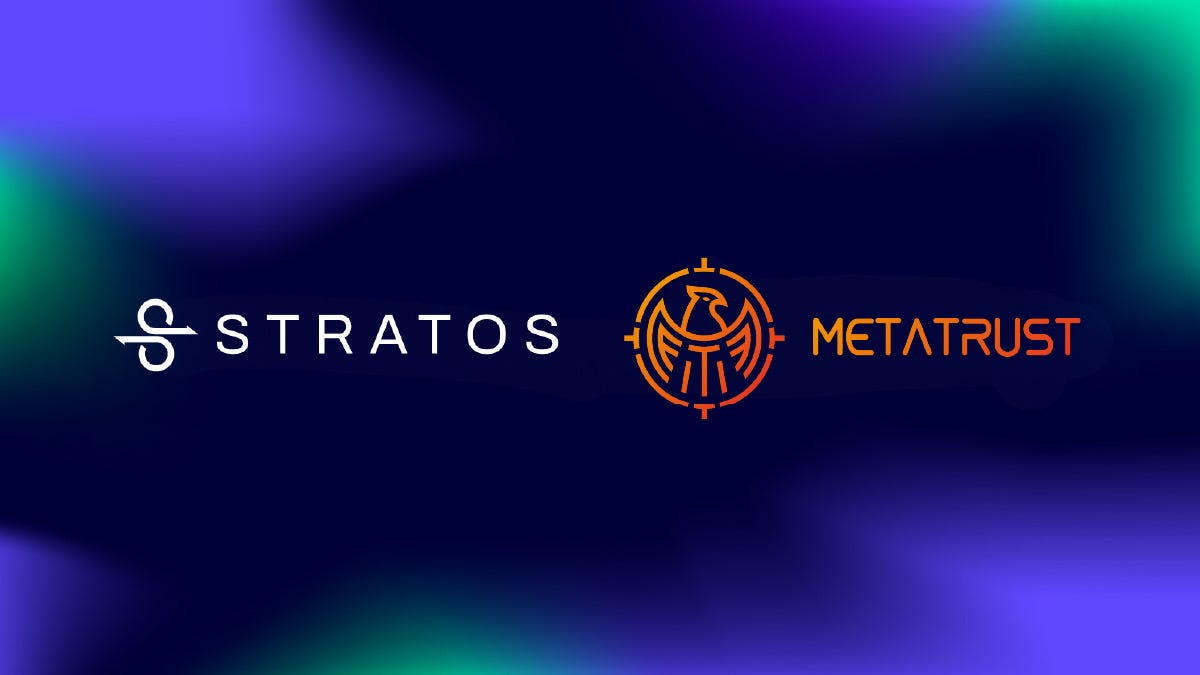
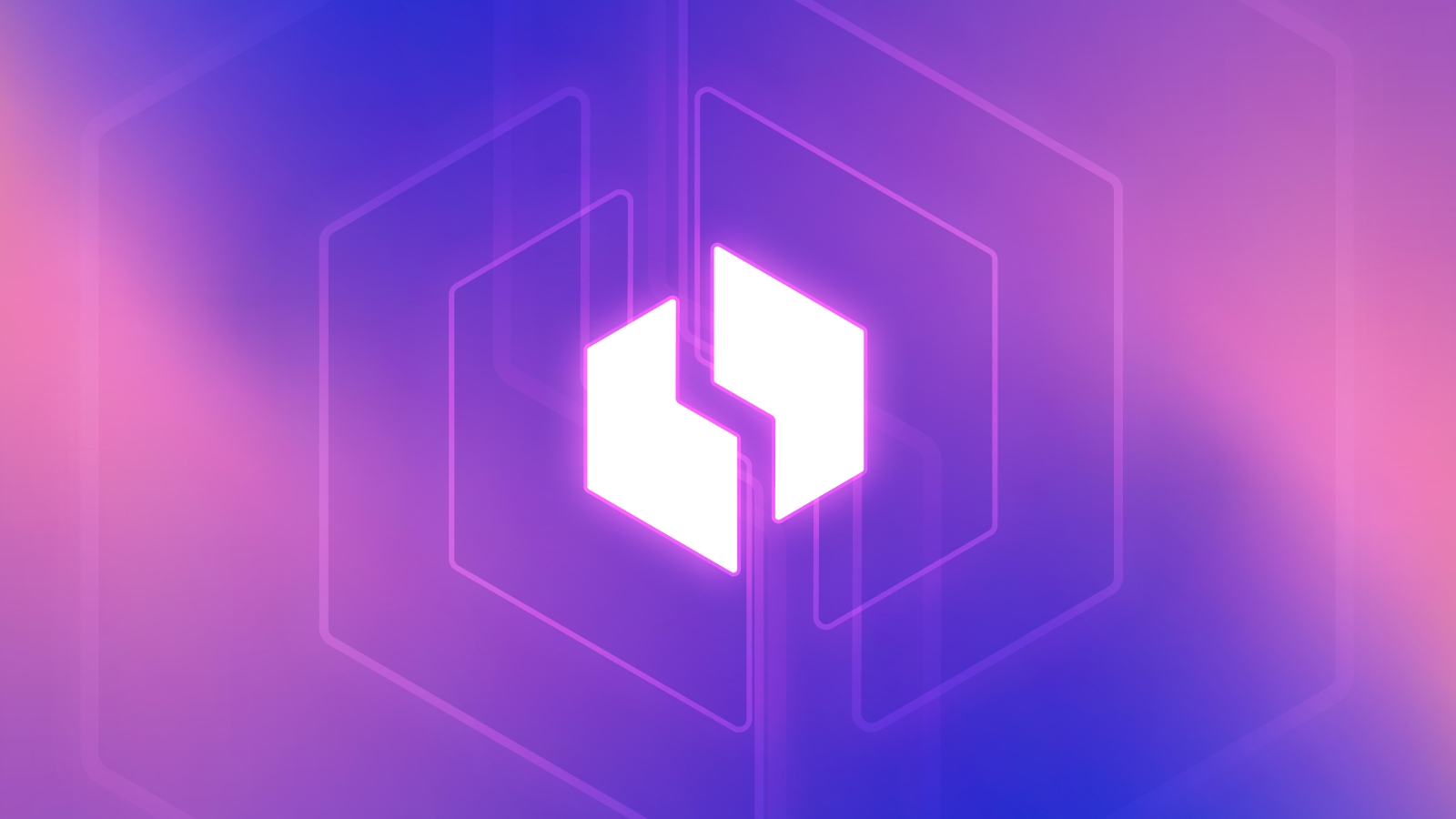
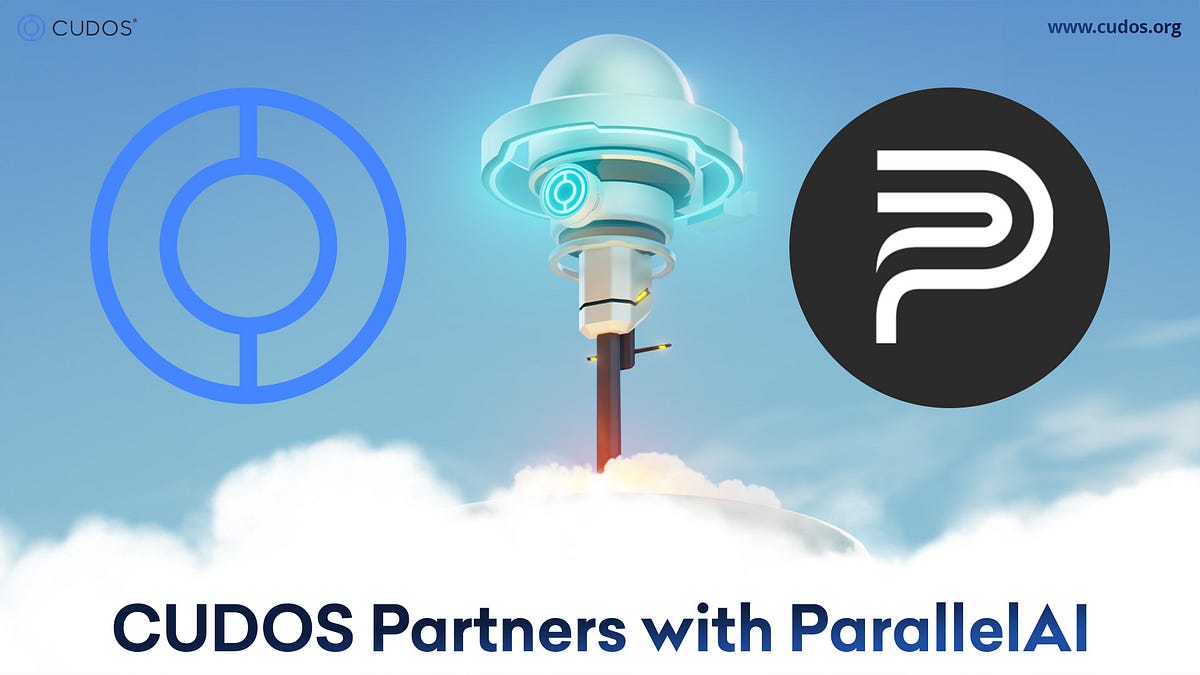