DePINエクスプローラー - DePIN Scan
トレンド 🔥
DePINの時価総額
$27,927,169,073
-0.6%
ボリューム
$11,276,084,035
-1.4%
DePINプロジェクト
296
DePINデバイス
19,074,201
+0.1%
DePINプロジェクト
DePIN Scanは、DePIN暗号プロジェクトのエクスプローラーです。合計296のDePINプロジェクトがあり、その総DePIN市場価値は$27,927,169,073で、総DePINデバイス数は19,074,201です。以下のプロジェクトをクリックして、今日からパッシブインカムを得る方法を学びましょう。
プロジェクト | トークン | カテゴリー | ソーシャルフォロワー | 時価総額 | トークン価格 | 24時間取引ボリューム | 1日 | 7日 | 30日 | 総デバイス数 | 過去7日間 |
---|---|---|---|---|---|---|---|---|---|---|---|
SOL | Chain | 2,857,235 | $120,883,280,379 | $254.77 | $9,967,258,528 | -0.4% | +20.5% | +49.0% | - | ||
![]() | RNDR | ServerAI | 205,471 | $3,741,439,821 | $7.23 | $339,291,368 | -2.3% | +9.1% | +46.1% | - | |
![]() | FIL | Server | 664,476 | $2,847,928,739 | $4.74 | $483,662,286 | +5.1% | +13.6% | +30.8% | 3,539 | |
![]() | THETA | ServerAI | 270,337 | $1,678,955,057 | $1.68 | $71,478,784 | -1.8% | +24.3% | +36.4% | 5,885 | |
![]() | AKT | ServerAI | 119,054 | $917,166,002 | $3.7 | $28,873,798 | -1.6% | +18.5% | +53.5% | 472 | |
![]() | HNT | Wireless | 213,792 | $911,965,355 | $5.28 | $20,042,493 | -6.6% | -8.8% | -23.5% | - | |
![]() | GRASS | ComputeAI | 514,215 | $504,085,993 | $2.07 | $212,395,363 | -14.2% | -23.6% | - | - | |
IOTX | Chain | 300,755 | $389,574,335 | $0.04126 | $24,510,431 | -2.0% | +7.9% | +7.0% | - | ||
![]() | IO | ComputeAI | 506,482 | $291,443,550 | $2.42 | $174,321,547 | +10.6% | +18.5% | +38.2% | - | |
![]() | NOS | ComputeAI | 59,263 | $266,524,857 | $3.19 | $3,596,360 | -11.4% | -1.3% | +50.0% | - | |
![]() | ATH | Compute | 844,097 | $233,015,004 | $0.05746 | $53,195,002 | -3.8% | -4.7% | +16.6% | - | |
![]() | HONEY | SensorAI | 48,864 | $186,448,319 | $0.06344 | $1,043,659 | +1.1% | -9.7% | +21.8% | 8,037 |
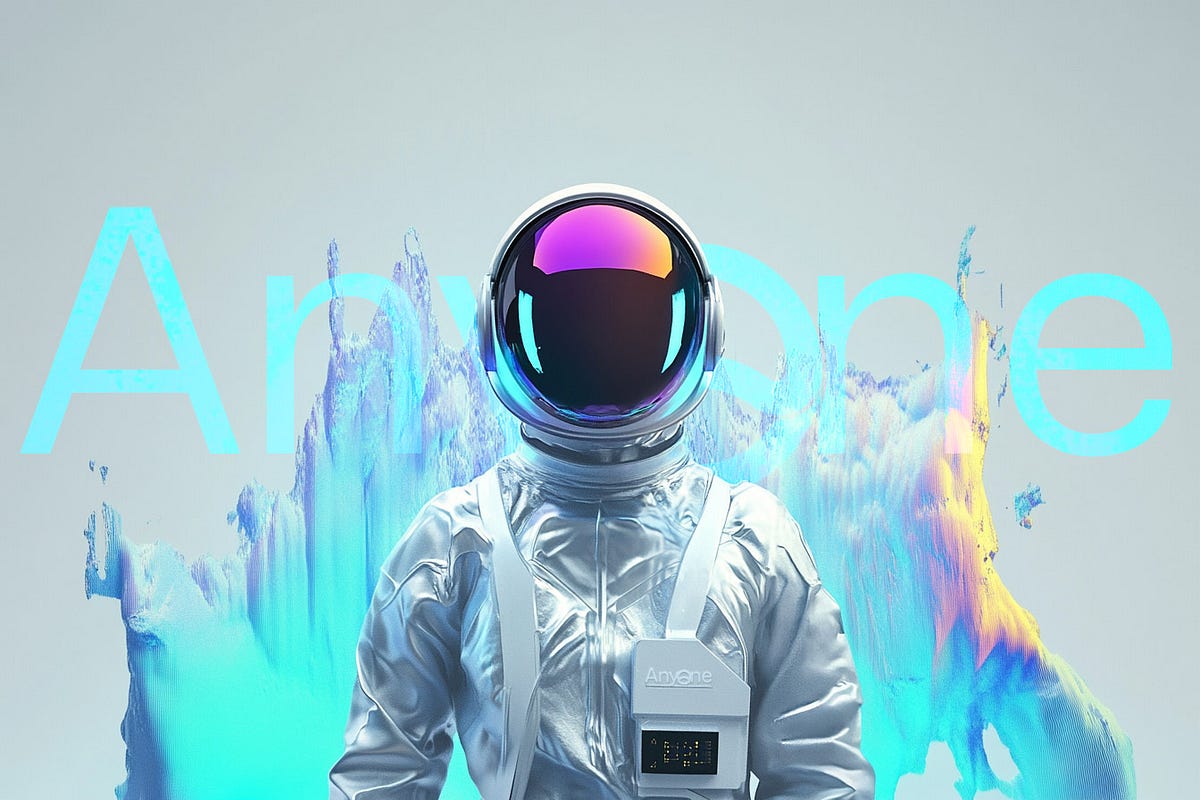
20時間前
ATOR's Journey: Building a Decentralized Future for Internet PrivacyA year ago, the Tor Project made a significant decision to remove all ATOR Relays from their network, which sparked a vision for a new decentralized future. Instead of surrendering, the team aimed to create a self-scaling, trustless network designed to enhance privacy for users worldwide. Their goal is to disrupt the VPN market by combining speed, reliability, and user-friendliness through bespoke hardware, ultimately making private browsing accessible to billions. This vision has now materialized with the launch of the Anyone Network, which boasts an impressive 5000 active relays, establishing itself as one of the largest and fastest mixnets globally.
Reflecting on the past year, the team expresses gratitude to their community for their unwavering support during challenging times. Despite the difficulties, the project demonstrated resilience and strength, showcasing the power of decentralization and community alignment. The leadership and engineering teams remained intact throughout the transition, empowering former community members to take on more significant roles within the ecosystem. The project has evolved into an open-source initiative, expanding from a single Smartweave registration protocol to over 30 active repositories contributing to blockchain technology, privacy, hardware, and applications.
Looking ahead, the team acknowledges the journey is far from over, with plans to further decentralize the network following the transition to their AO distribution protocol. They emphasize that community governance will play a crucial role in maintaining the network's safety and performance. The spirit of ATOR is now emblematic of overcoming setbacks in the Web3 space, and the team is committed to transparency through regular updates. As they tackle the larger challenge of global privacy adoption, they remain dedicated to building a Web3 ecosystem that has the potential to transform lives.
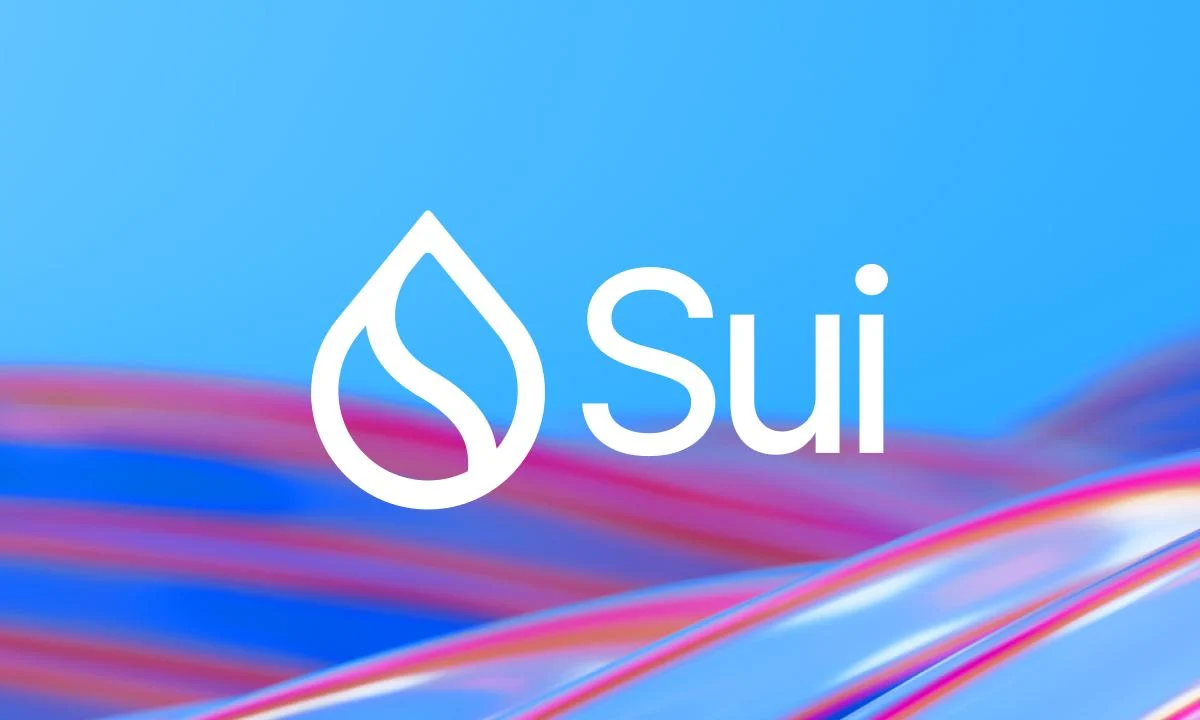
20時間前
Sui財団がフランクリン・テンプルトンと提携し、ブロックチェーンの革新を促進2024年11月22日、Sui財団はフランクリン・テンプルトンデジタルアセットと戦略的パートナーシップを発表し、Suiブロックチェーンエコシステム内での革新を推進することを目指しています。このコラボレーションは、ブロックチェーンベースのソリューションの開発者に価値の機会を創出することにより、Layer 1およびスマートコントラクトプラットフォームであるSuiの開発を強化することを目的としています。Suiのエコシステム開発責任者であるジャミール・カルファンは、このパートナーシップがフランクリン・テンプルトンが取り組んでいる分散型金融の課題に触発されたSuiの技術の検証であると強調しました。
フランクリン・テンプルトンデジタルアセットは、数年間にわたりブロックチェーン技術に積極的に関与しており、ソリューションの構築、ノードバリデーターの運営、投資戦略の開発に焦点を当てています。彼らの専任のデジタル資産研究チームは、トークノミクス分析とデータサイエンスの洞察を活用して、製品開発や投資判断を導いています。フランクリン・テンプルトンのデジタル資産管理担当SVPであるトニー・ペコーレは、Suiチームが進めている進展に興奮を表明し、ブロックチェーン技術は関心が高まる一方で、技術的な制約に直面することが多いと指摘しました。
Suiエコシステムには、中央集権的な注文書に似た分散型金融ソリューションであるDeepBookや、分散型モバイルネットワークをサポートするKarrier Oneなどの革新的なプロジェクトがすでに存在しています。さらに、Suiは最近、ネイティブUSDCを立ち上げ、ユーザーに広く使用されているステーブルコインへの直接アクセスを提供し、ブリッジされた資産に関連するリスクを軽減しています。安全でスケーラブルなプラットフォームとして、Suiはゲーム、DeFi、トークン化された証券など、さまざまな分野のアプリケーション開発者にとっての主要な目的地としての地位を確立しています。
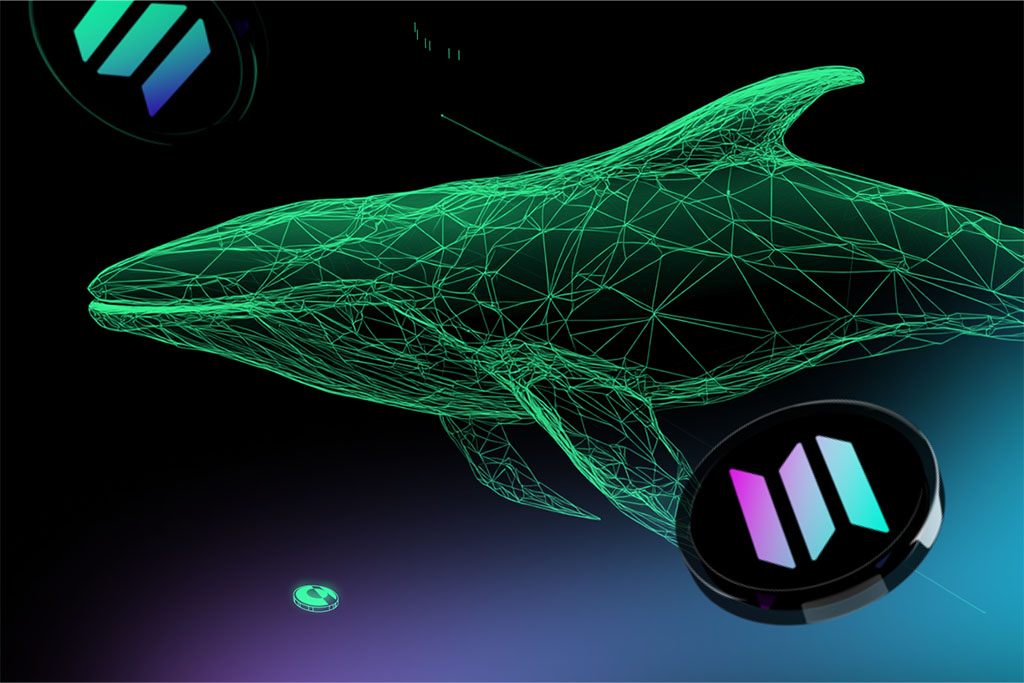
20時間前
AkashネットワークとLunexネットワークが暗号通貨の革新をリード暗号通貨市場は重要な発展を遂げており、特にAkashネットワークのDePINプロトコルが最近、10億ドルの時価総額を超えたことが注目されています。この急増はAkashの価格の顕著な上昇をもたらし、投資家の信頼が高まっていることを反映しています。一方、Solanaは新たな最高値(ATH)を達成する寸前であり、その時価総額の流入によりBNBを超えることが可能になっています。Solanaの迫るATHに対する興奮は、プラットフォームにとっての勝利だけでなく、より広範な暗号通貨エコシステムにとっても勝利であり、主流の採用と堅実な開発活動の可能性を示しています。
分散型金融(DeFi)の分野では、Lunexネットワークが有望な新しいプレーヤーとして登場しました。このハイブリッド取引所は、50,000以上の取引ペアと手数料ゼロの構造を提供することで、クロスチェーントレーディングを促進するユニークなソリューションを提供しています。Lunexネットワークは、初心者と経験豊富なトレーダーの両方にとってDeFiを簡素化し、小売投資家にとってよりアクセスしやすくすることを目指しています。すでに2.95百万ドルの印象的な流動性を確保しているLunexは、DeFi分野での重要なファシリテーターとしての地位を確立し、この魅力的な市場へのアクセスを民主化する可能性があります。
Akashネットワーク、Solana、Lunexネットワークの台頭は、従来の金融を超えた分散型革新への広範なトレンドを浮き彫りにしています。過去24時間でのAkashの10%の急増と、過去1週間での36%の驚異的な増加は、従来のクラウドサービスを破壊する可能性を強調しています。分散型で信頼のないプラットフォームへの需要が高まる中、これらのトークンは魅力的な投資機会を提供しています。SolanaがATHに近づき、Akashの勢いが続く中、投資家はこれらの動向を注視しており、LunexネットワークはそのDeFiオファリングで引き続き注目を集めています。
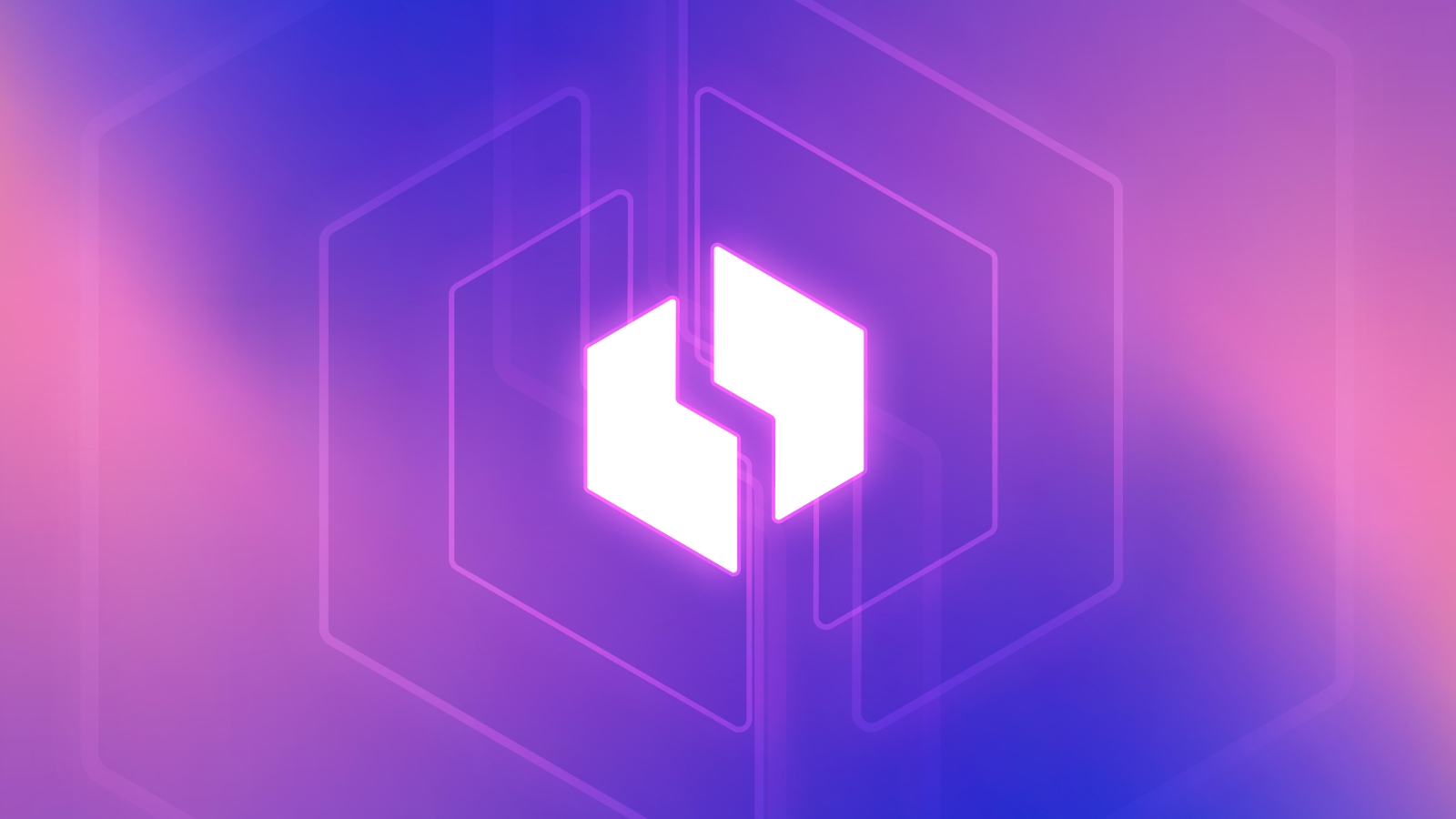
20時間前
Enhancing Context Recall in Retrieval-Augmented GenerationRetrieval-augmented generation (RAG) has emerged as a pivotal method for integrating large language models (LLMs) into specialized business applications, enabling the infusion of proprietary data into model responses. Despite its effectiveness during the proof of concept (POC) phase, developers often face significant accuracy drops when transitioning RAG into production. This issue is particularly pronounced during the retrieval phase, where the aim is to accurately fetch the most relevant context for a given query, a metric known as context recall. This article delves into strategies for enhancing context recall by customizing and fine-tuning embedding models, ultimately improving RAG's performance in real-world applications.
RAG operates in two main steps: retrieval and generation. In the retrieval phase, the model converts text into vectors, indexes, retrieves, and re-ranks these vectors to identify the top matches. However, failures in this phase can lead to missed relevant contexts, resulting in lower context recall and less accurate generation outputs. One effective solution is to adapt the embedding model, which is designed to understand relationships between text data, to produce embeddings that are specific to the dataset being used. This fine-tuning allows the model to generate similar vectors for similar sentences, enhancing its ability to retrieve context that is highly relevant to the query.
To improve context recall, it is essential to prepare a tailored dataset that reflects the types of queries the model will encounter. This involves extracting a diverse range of questions from the knowledge base, paraphrasing them for variability, and organizing them by relevance. Additionally, constructing an evaluation dataset helps assess the model's performance in a realistic setting. By employing an Information Retrieval Evaluator, developers can measure metrics like Recall@k and Precision@k to gauge retrieval accuracy. Ultimately, fine-tuning the embedding model can lead to substantial improvements in context recall, ensuring that RAG remains accurate and reliable in production environments.