DePIN 探索者 - DePIN Scan
趨勢 🔥
DePIN 市值
$27,927,169,073
-0.6%
交易量
$11,276,084,035
-1.4%
DePIN項目
296
DePIN設備
19,074,201
+0.1%
DePIN 項目
DePIN Scan是DePIN加密項目的探索器。有296個DePIN項目,總共的DePIN市值為$27,927,169,073,並且擁有19,074,201台DePIN設備。點擊下方的項目以了解如何從今天開始賺取被動收入。
項目 | 代幣 | 類別 | 社交跟隨者 | 市值 | 代幣價格 | 24 小時交易量 | 1 天 | 7 天 | 30 天 | 設備總數 | 最近 7 天 |
---|---|---|---|---|---|---|---|---|---|---|---|
SOL | Chain | 2,857,235 | $120,864,631,212 | $254.84 | $9,724,273,028 | -0.4% | +20.5% | +49.0% | - | ||
![]() | RNDR | ServerAI | 205,471 | $3,742,903,320 | $7.25 | $340,029,884 | -2.3% | +9.1% | +46.1% | - | |
![]() | FIL | Server | 664,476 | $2,855,809,767 | $4.76 | $487,055,490 | +5.1% | +13.6% | +30.8% | 3,539 | |
![]() | THETA | ServerAI | 270,337 | $1,682,594,695 | $1.68 | $71,461,361 | -1.8% | +24.3% | +36.4% | 5,885 | |
![]() | AKT | ServerAI | 119,054 | $916,751,087 | $3.71 | $28,967,583 | -1.6% | +18.5% | +53.5% | 472 | |
![]() | HNT | Wireless | 213,792 | $911,135,341 | $5.29 | $20,049,936 | -6.6% | -8.8% | -23.5% | - | |
![]() | GRASS | ComputeAI | 514,215 | $509,003,367 | $2.1 | $214,017,941 | -14.2% | -23.6% | - | - | |
IOTX | Chain | 300,755 | $389,768,899 | $0.04133 | $24,240,834 | -2.0% | +7.9% | +7.0% | - | ||
![]() | IO | ComputeAI | 506,482 | $291,275,383 | $2.42 | $176,306,732 | +10.6% | +18.5% | +38.2% | - | |
![]() | NOS | ComputeAI | 59,263 | $266,826,358 | $3.2 | $3,561,368 | -11.4% | -1.3% | +50.0% | - | |
![]() | ATH | Compute | 844,097 | $233,164,142 | $0.05750 | $53,017,014 | -3.8% | -4.7% | +16.6% | - | |
![]() | HONEY | SensorAI | 48,864 | $186,302,000 | $0.06359 | $1,031,739 | +1.1% | -9.7% | +21.8% | 8,037 |
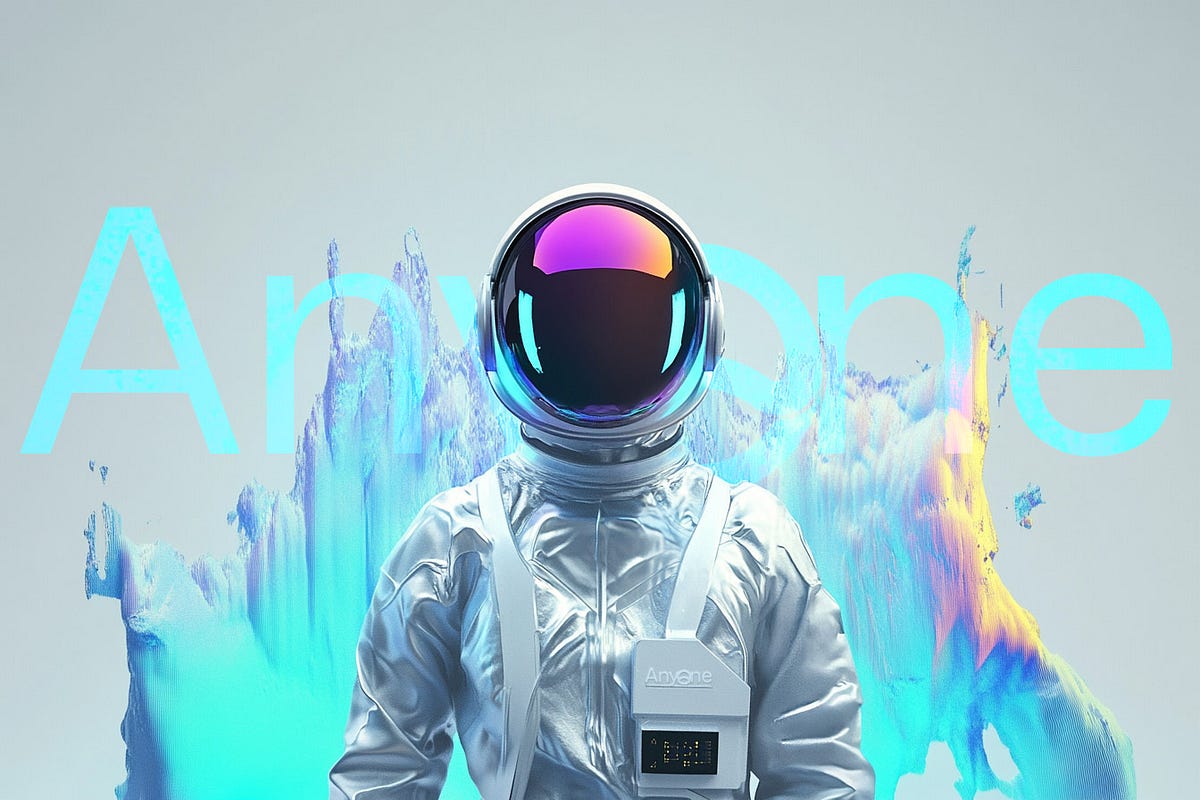
21 小時前
ATOR's Journey: Building a Decentralized Future for Internet PrivacyA year ago, the Tor Project made a significant decision to remove all ATOR Relays from their network, which sparked a vision for a new decentralized future. Instead of surrendering, the team aimed to create a self-scaling, trustless network designed to enhance privacy for users worldwide. Their goal is to disrupt the VPN market by combining speed, reliability, and user-friendliness through bespoke hardware, ultimately making private browsing accessible to billions. This vision has now materialized with the launch of the Anyone Network, which boasts an impressive 5000 active relays, establishing itself as one of the largest and fastest mixnets globally.
Reflecting on the past year, the team expresses gratitude to their community for their unwavering support during challenging times. Despite the difficulties, the project demonstrated resilience and strength, showcasing the power of decentralization and community alignment. The leadership and engineering teams remained intact throughout the transition, empowering former community members to take on more significant roles within the ecosystem. The project has evolved into an open-source initiative, expanding from a single Smartweave registration protocol to over 30 active repositories contributing to blockchain technology, privacy, hardware, and applications.
Looking ahead, the team acknowledges the journey is far from over, with plans to further decentralize the network following the transition to their AO distribution protocol. They emphasize that community governance will play a crucial role in maintaining the network's safety and performance. The spirit of ATOR is now emblematic of overcoming setbacks in the Web3 space, and the team is committed to transparency through regular updates. As they tackle the larger challenge of global privacy adoption, they remain dedicated to building a Web3 ecosystem that has the potential to transform lives.
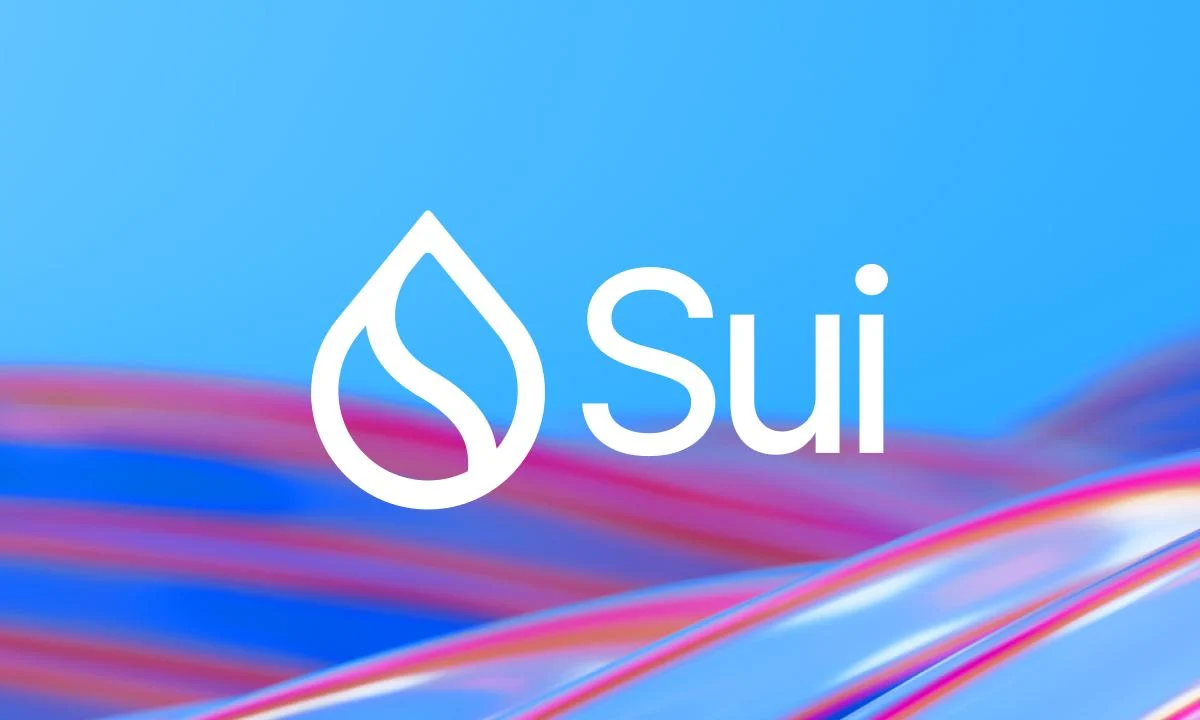
21 小時前
Sui Foundation Partners with Franklin Templeton to Enhance Blockchain InnovationOn November 22, 2024, the Sui Foundation announced a strategic partnership with Franklin Templeton Digital Assets, aimed at driving innovation within the Sui blockchain ecosystem. This collaboration is set to enhance the development of Sui, a Layer 1 and smart contract platform, by creating value opportunities for builders of blockchain-based solutions. Jameel Khalfan, Head of Ecosystem Development at Sui, highlighted that the partnership is a validation of Sui's technology, which was inspired by challenges in decentralized finance that Franklin Templeton is addressing.
Franklin Templeton Digital Assets has been actively involved in blockchain technology for several years, focusing on building solutions, running node validators, and developing investment strategies. Their dedicated digital assets research team employs tokenomic analysis and data science insights to guide product development and investment decisions. Tony Pecore, SVP and Director of Digital Asset Management at Franklin Templeton, expressed excitement about the advancements being made by the Sui team, noting that blockchain technology has often faced technical limitations despite its growing interest.
The Sui ecosystem is already home to innovative projects such as DeepBook, a decentralized finance solution akin to a central limit order book, and Karrier One, which supports a decentralized mobile network. Additionally, Sui has recently launched native USDC, providing users with direct access to a widely used stablecoin while mitigating risks associated with bridged assets. As a secure and scalable platform, Sui is positioning itself as a premier destination for application builders across various sectors, including gaming, DeFi, and tokenized securities.
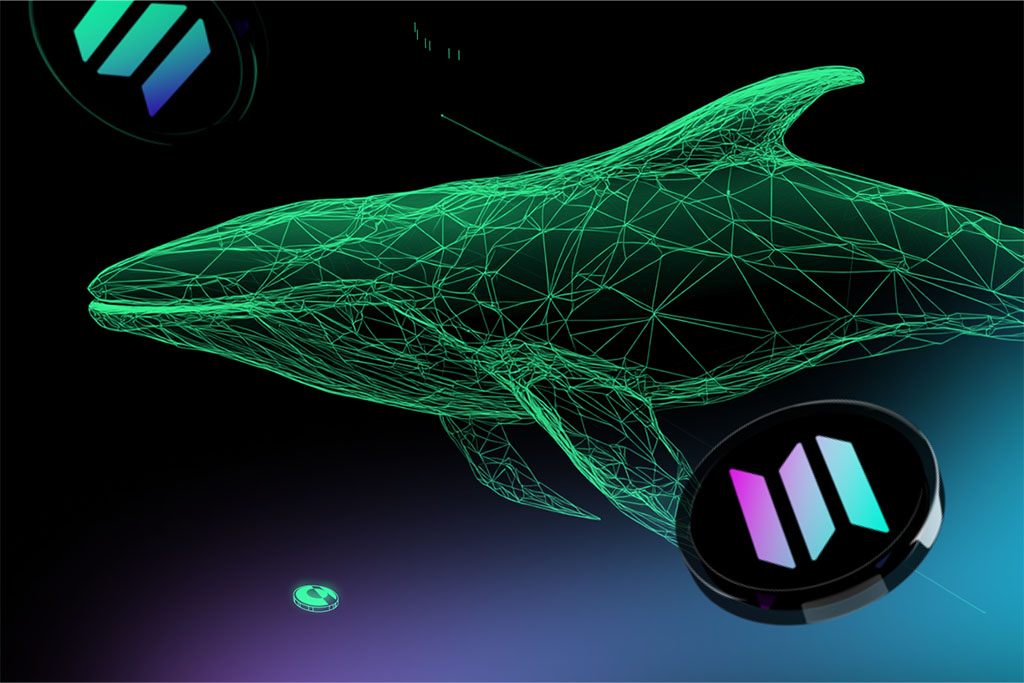
21 小時前
Akash 網絡和 Lunex 網絡引領加密貨幣創新加密貨幣市場正在見證重大發展,特別是 Akash 網絡的 DePIN 協議,最近已經突破十億美元的市值。這一激增導致 Akash 價格顯著上漲,反映出投資者信心的增強。與此同時,Solana 正在邊緣達成新的歷史高點 (ATH),其市值流入使其超越 BNB。圍繞 Solana 即將達成的 ATH 的興奮不僅是該平台的勝利,也是整個加密貨幣生態系統的勝利,展示了其主流採用和強勁發展活動的潛力。
在去中心化金融 (DeFi) 領域,Lunex 網絡已經成為一個有前途的新參與者。這個混合交易所通過提供超過 50,000 個交易對和零手續費結構來促進跨鏈交易,提供了一個獨特的解決方案。Lunex 網絡旨在簡化 DeFi,讓新手和經驗豐富的交易者都能更輕鬆地進入,讓零售投資者更容易接觸。Lunex 已經確保了 295 萬美元的驚人流動性,正將自己定位為 DeFi 領域的關鍵促進者,可能會使這個利潤豐厚的市場變得更加民主化。
Akash 網絡、Solana 和 Lunex 網絡的崛起突顯了超越傳統金融的去中心化創新的更廣泛趨勢。Akash 最近在過去 24 小時內上漲 10% 和在過去一周內顯著上漲 36% 強調了其顛覆傳統雲服務的潛力。隨著對去中心化、無需信任的平台需求的增長,這些代幣提供了令人興奮的投資機會。隨著 Solana 接近其 ATH 和 Akash 的動力,投資者正密切關注這些發展,而 Lunex 網絡則繼續以其 DeFi 產品吸引關注。
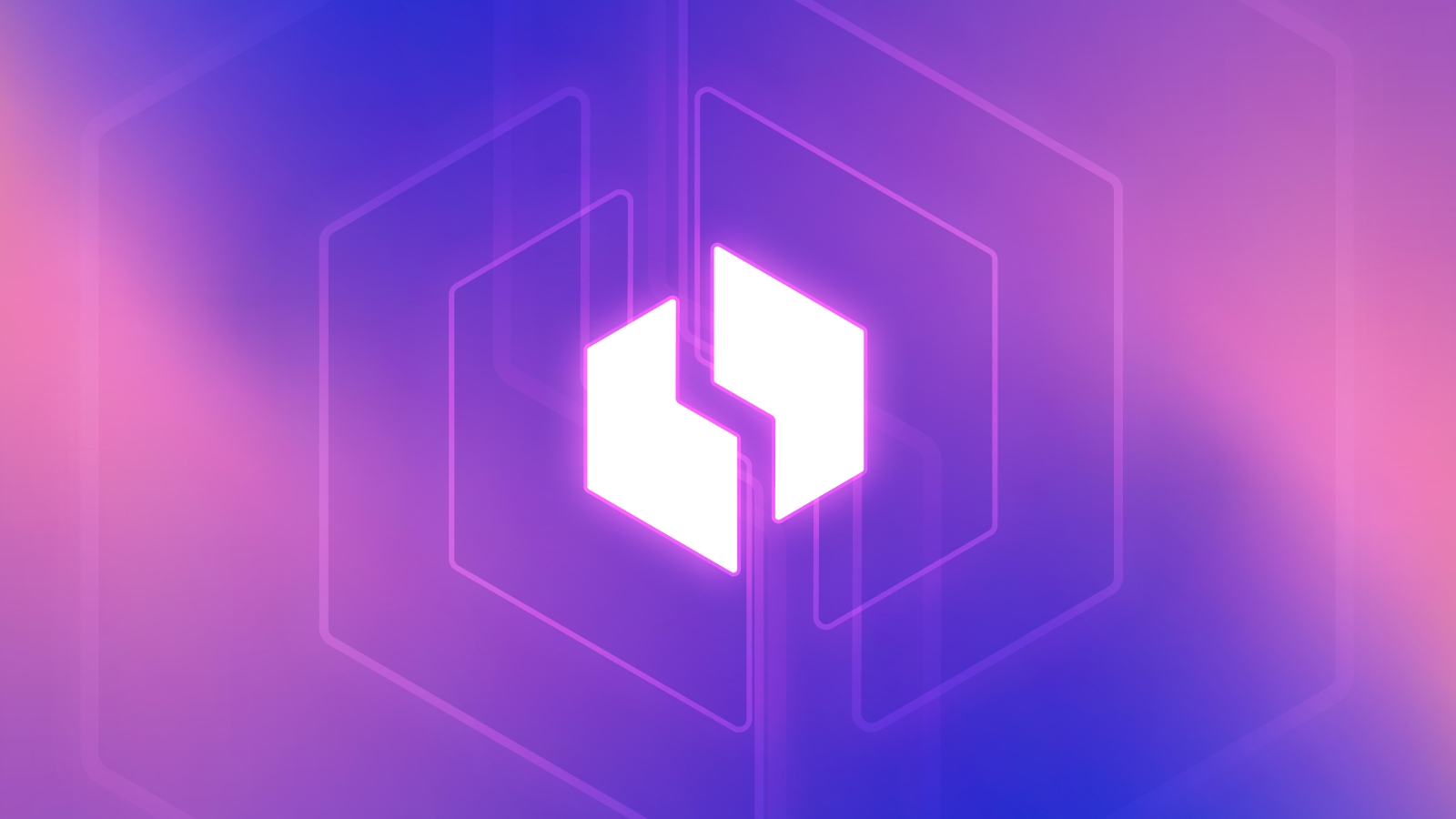
21 小時前
Enhancing Context Recall in Retrieval-Augmented GenerationRetrieval-augmented generation (RAG) has emerged as a pivotal method for integrating large language models (LLMs) into specialized business applications, enabling the infusion of proprietary data into model responses. Despite its effectiveness during the proof of concept (POC) phase, developers often face significant accuracy drops when transitioning RAG into production. This issue is particularly pronounced during the retrieval phase, where the aim is to accurately fetch the most relevant context for a given query, a metric known as context recall. This article delves into strategies for enhancing context recall by customizing and fine-tuning embedding models, ultimately improving RAG's performance in real-world applications.
RAG operates in two main steps: retrieval and generation. In the retrieval phase, the model converts text into vectors, indexes, retrieves, and re-ranks these vectors to identify the top matches. However, failures in this phase can lead to missed relevant contexts, resulting in lower context recall and less accurate generation outputs. One effective solution is to adapt the embedding model, which is designed to understand relationships between text data, to produce embeddings that are specific to the dataset being used. This fine-tuning allows the model to generate similar vectors for similar sentences, enhancing its ability to retrieve context that is highly relevant to the query.
To improve context recall, it is essential to prepare a tailored dataset that reflects the types of queries the model will encounter. This involves extracting a diverse range of questions from the knowledge base, paraphrasing them for variability, and organizing them by relevance. Additionally, constructing an evaluation dataset helps assess the model's performance in a realistic setting. By employing an Information Retrieval Evaluator, developers can measure metrics like Recall@k and Precision@k to gauge retrieval accuracy. Ultimately, fine-tuning the embedding model can lead to substantial improvements in context recall, ensuring that RAG remains accurate and reliable in production environments.