DePIN 탐색기 - DePIN Scan
인기 🔥
DePIN 시가총액
$27,927,169,073
-0.6%
거래량
$11,276,084,035
-1.4%
DePIN 프로젝트
296
DePIN 장치
19,074,201
+0.1%
DePIN 프로젝트
DePIN Scan은 DePIN 암호화폐 프로젝트를 탐색하는 도구입니다. 총 296개의 DePIN 프로젝트가 있으며, 이들의 합산된 DePIN 시장 규모는 $27,927,169,073이고, 전체 DePIN 장치 수는 19,074,201개 입니다. 아래의 프로젝트를 클릭하여 오늘부터 수동 소득을 얻기 시작하는 방법을 배워보세요.
프로젝트 | 토큰 | 카테고리 | 소셜 팔로워 | 시가총액 | 토큰 가격 | 24시간 거래량 | 1일 | 7일 | 30일 | 총 장치 | 최근 7일 |
---|---|---|---|---|---|---|---|---|---|---|---|
SOL | Chain | 2,857,235 | $120,864,631,212 | $254.77 | $9,971,458,756 | -0.4% | +20.5% | +49.0% | - | ||
![]() | RNDR | ServerAI | 205,471 | $3,742,903,320 | $7.24 | $339,376,716 | -2.3% | +9.1% | +46.1% | - | |
![]() | FIL | Server | 664,476 | $2,855,809,767 | $4.75 | $484,952,421 | +5.1% | +13.6% | +30.8% | 3,539 | |
![]() | THETA | ServerAI | 270,337 | $1,681,150,458 | $1.68 | $71,805,048 | -1.8% | +24.3% | +36.4% | 5,885 | |
![]() | AKT | ServerAI | 119,054 | $916,751,087 | $3.71 | $28,950,611 | -1.6% | +18.5% | +53.5% | 472 | |
![]() | HNT | Wireless | 213,792 | $911,135,341 | $5.28 | $20,036,141 | -6.6% | -8.8% | -23.5% | - | |
![]() | GRASS | ComputeAI | 514,215 | $509,003,367 | $2.09 | $213,338,770 | -14.2% | -23.6% | - | - | |
IOTX | Chain | 300,755 | $389,768,899 | $0.04129 | $24,247,785 | -2.0% | +7.9% | +7.0% | - | ||
![]() | IO | ComputeAI | 506,482 | $291,261,056 | $2.42 | $176,155,909 | +10.6% | +18.5% | +38.2% | - | |
![]() | NOS | ComputeAI | 59,263 | $266,826,358 | $3.2 | $3,561,709 | -11.4% | -1.3% | +50.0% | - | |
![]() | ATH | Compute | 844,097 | $233,164,142 | $0.05752 | $53,059,398 | -3.8% | -4.7% | +16.6% | - | |
![]() | HONEY | SensorAI | 48,864 | $186,233,717 | $0.06343 | $1,030,376 | +1.1% | -9.7% | +21.8% | 8,037 |
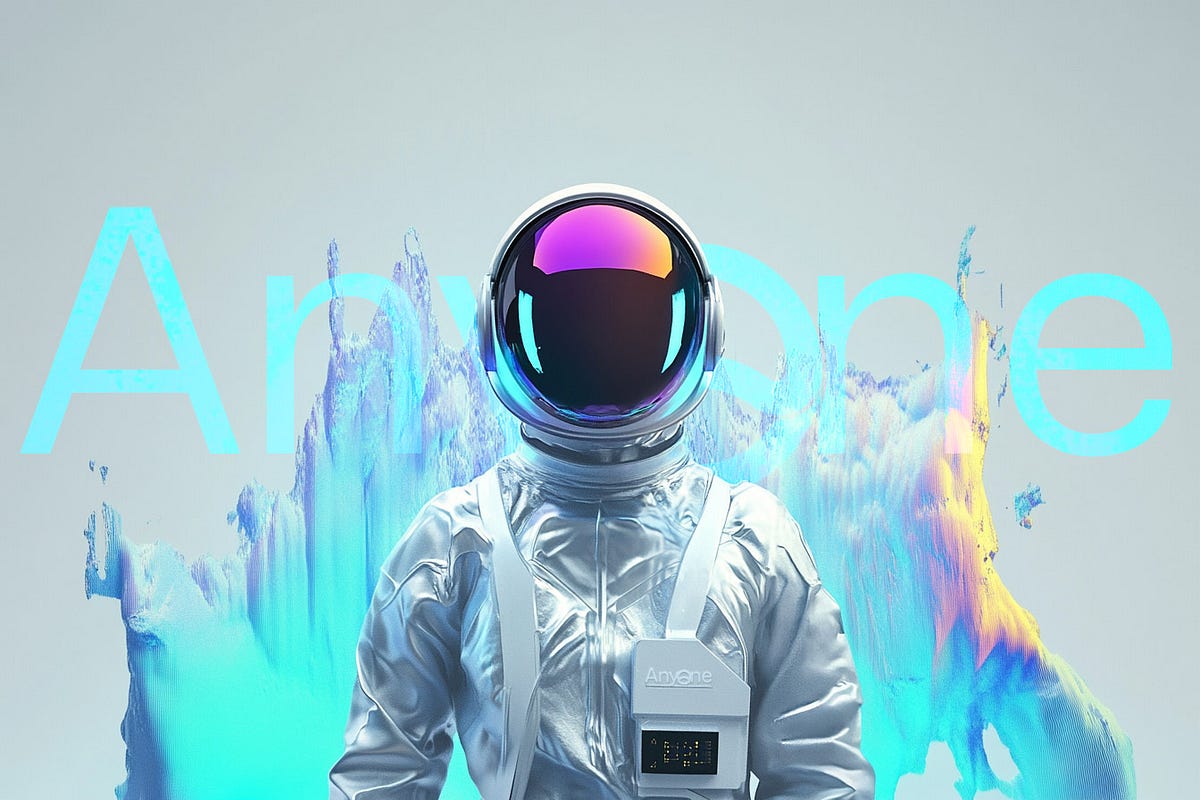
20시간 전
ATOR's Journey: Building a Decentralized Future for Internet PrivacyA year ago, the Tor Project made a significant decision to remove all ATOR Relays from their network, which sparked a vision for a new decentralized future. Instead of surrendering, the team aimed to create a self-scaling, trustless network designed to enhance privacy for users worldwide. Their goal is to disrupt the VPN market by combining speed, reliability, and user-friendliness through bespoke hardware, ultimately making private browsing accessible to billions. This vision has now materialized with the launch of the Anyone Network, which boasts an impressive 5000 active relays, establishing itself as one of the largest and fastest mixnets globally.
Reflecting on the past year, the team expresses gratitude to their community for their unwavering support during challenging times. Despite the difficulties, the project demonstrated resilience and strength, showcasing the power of decentralization and community alignment. The leadership and engineering teams remained intact throughout the transition, empowering former community members to take on more significant roles within the ecosystem. The project has evolved into an open-source initiative, expanding from a single Smartweave registration protocol to over 30 active repositories contributing to blockchain technology, privacy, hardware, and applications.
Looking ahead, the team acknowledges the journey is far from over, with plans to further decentralize the network following the transition to their AO distribution protocol. They emphasize that community governance will play a crucial role in maintaining the network's safety and performance. The spirit of ATOR is now emblematic of overcoming setbacks in the Web3 space, and the team is committed to transparency through regular updates. As they tackle the larger challenge of global privacy adoption, they remain dedicated to building a Web3 ecosystem that has the potential to transform lives.
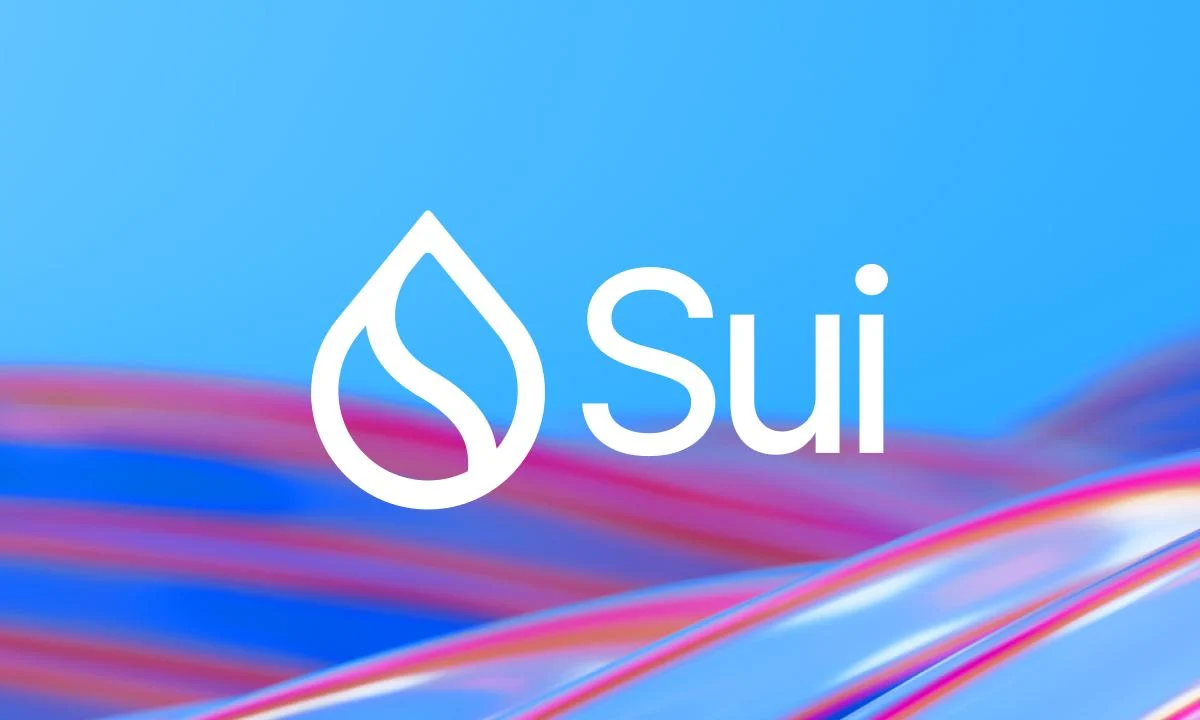
20시간 전
Sui 재단, 블록체인 혁신 강화를 위해 프랭클린 템플턴과 파트너십 체결2024년 11월 22일, Sui 재단은 Sui 블록체인 생태계 내 혁신을 촉진하기 위해 프랭클린 템플턴 디지털 자산과 전략적 파트너십을 발표했습니다. 이 협력은 블록체인 기반 솔루션의 제작자들에게 가치 기회를 창출함으로써 Sui, 레이어 1 및 스마트 계약 플랫폼의 개발을 강화할 것입니다. Sui의 생태계 개발 책임자인 자밀 칼판은 이 파트너십이 프랭클린 템플턴이 해결하고 있는 탈중앙화 금융의 도전에서 영감을 받은 Sui 기술의 검증이라고 강조했습니다.
프랭클린 템플턴 디지털 자산은 여러 해 동안 블록체인 기술에 적극적으로 참여해 왔으며, 솔루션 구축, 노드 검증자 운영 및 투자 전략 개발에 중점을 두고 있습니다. 그들의 전담 디지털 자산 연구팀은 토큰 경제 분석 및 데이터 과학 통찰력을 활용하여 제품 개발 및 투자 결정을 안내합니다. 프랭클린 템플턴의 디지털 자산 관리 부사장 겸 이사인 토니 페코레는 Sui 팀이 이루고 있는 발전에 대해 흥분을 표하며, 블록체인 기술이 관심이 커지고 있음에도 불구하고 종종 기술적 한계에 직면해 있다고 언급했습니다.
Sui 생태계는 중앙 집중식 제한 주문서와 유사한 탈중앙화 금융 솔루션인 DeepBook과 탈중앙화 모바일 네트워크를 지원하는 Karrier One과 같은 혁신적인 프로젝트의 본거지입니다. 또한, Sui는 최근 네이티브 USDC를 출시하여 사용자에게 널리 사용되는 스테이블코인에 직접 접근할 수 있는 기회를 제공하면서 브릿지 자산과 관련된 위험을 완화하고 있습니다. 안전하고 확장 가능한 플랫폼으로서 Sui는 게임, DeFi 및 토큰화된 증권을 포함한 다양한 분야의 애플리케이션 제작자들에게 주요 목적지로 자리매김하고 있습니다.
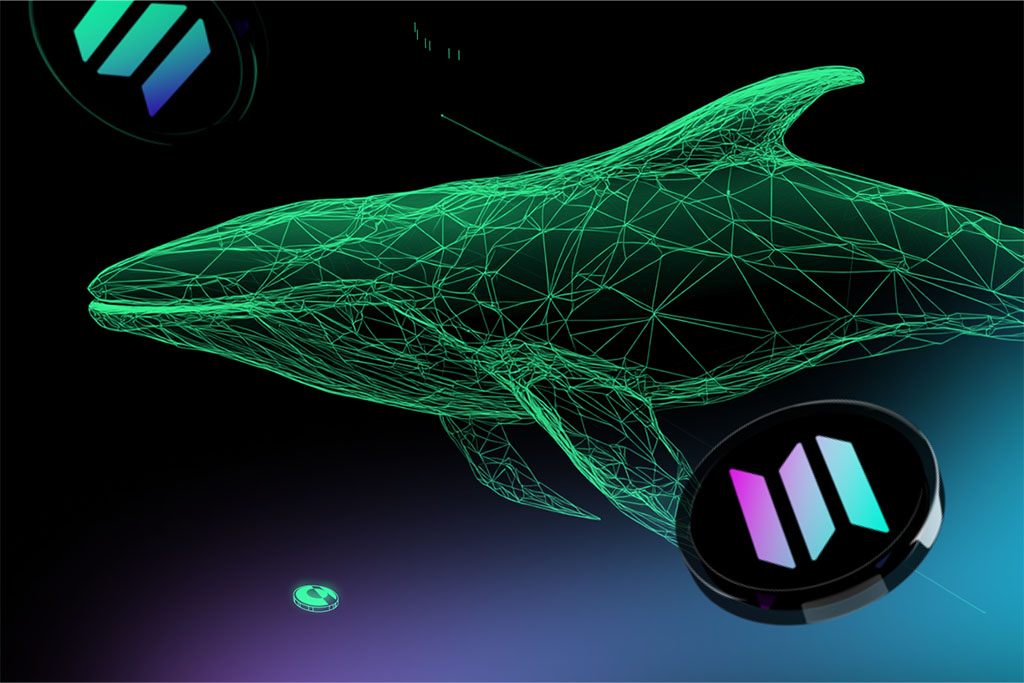
20시간 전
아카시 네트워크와 루넥스 네트워크, 암호화폐 혁신을 선도하다암호화폐 시장은 아카시 네트워크의 DePIN 프로토콜과 함께 중요한 발전을 목격하고 있으며, 최근 10억 달러의 시장 가치를 초과했습니다. 이 급증은 아카시 가격의 눈에 띄는 상승으로 이어져 투자자 신뢰가 커지고 있음을 반영합니다. 한편, 솔라나는 새로운 사상 최고치(ATH)에 도달할 직전이며, 시장 가치 유입으로 BNB를 초과할 수 있게 되었습니다. 솔라나의 임박한 ATH에 대한 기대감은 플랫폼에 대한 승리일 뿐만 아니라, 주류 채택과 강력한 개발 활동을 보여주는 광범위한 암호화폐 생태계에 대한 승리입니다.
탈중앙화 금융(DeFi) 분야에서 루넥스 네트워크는 유망한 새로운 플레이어로 떠올랐습니다. 이 하이브리드 거래소는 50,000개 이상의 거래 쌍과 수수료 없는 구조를 통해 크로스 체인 거래를 용이하게 하는 독특한 솔루션을 제공합니다. 루넥스 네트워크는 초보자와 경험이 풍부한 거래자 모두에게 DeFi를 간소화하여 소매 투자자에게 더 쉽게 접근할 수 있도록 하는 것을 목표로 하고 있습니다. 이미 확보된 295만 달러의 인상적인 유동성을 바탕으로, 루넥스는 DeFi 공간에서 주요 촉진자로 자리매김하고 있으며, 이 수익성 있는 시장에 대한 접근을 민주화할 가능성이 있습니다.
아카시 네트워크, 솔라나, 루넥스 네트워크의 상승은 전통 금융을 넘어선 탈중앙화 혁신에 대한 더 넓은 추세를 강조합니다. 아카시의 최근 24시간 동안 10% 상승과 지난 주 36%의 놀라운 증가율은 기존 클라우드 서비스에 대한 잠재적 혼란을 강조합니다. 탈중앙화되고 신뢰할 수 없는 플랫폼에 대한 수요가 증가함에 따라, 이러한 토큰은 흥미로운 투자 기회를 제공합니다. 솔라나가 ATH에 가까워지고 아카시의 모멘텀과 함께, 투자자들은 이러한 발전을 주의 깊게 지켜보고 있으며, 루넥스 네트워크는 DeFi 제공으로 계속해서 주목받고 있습니다.
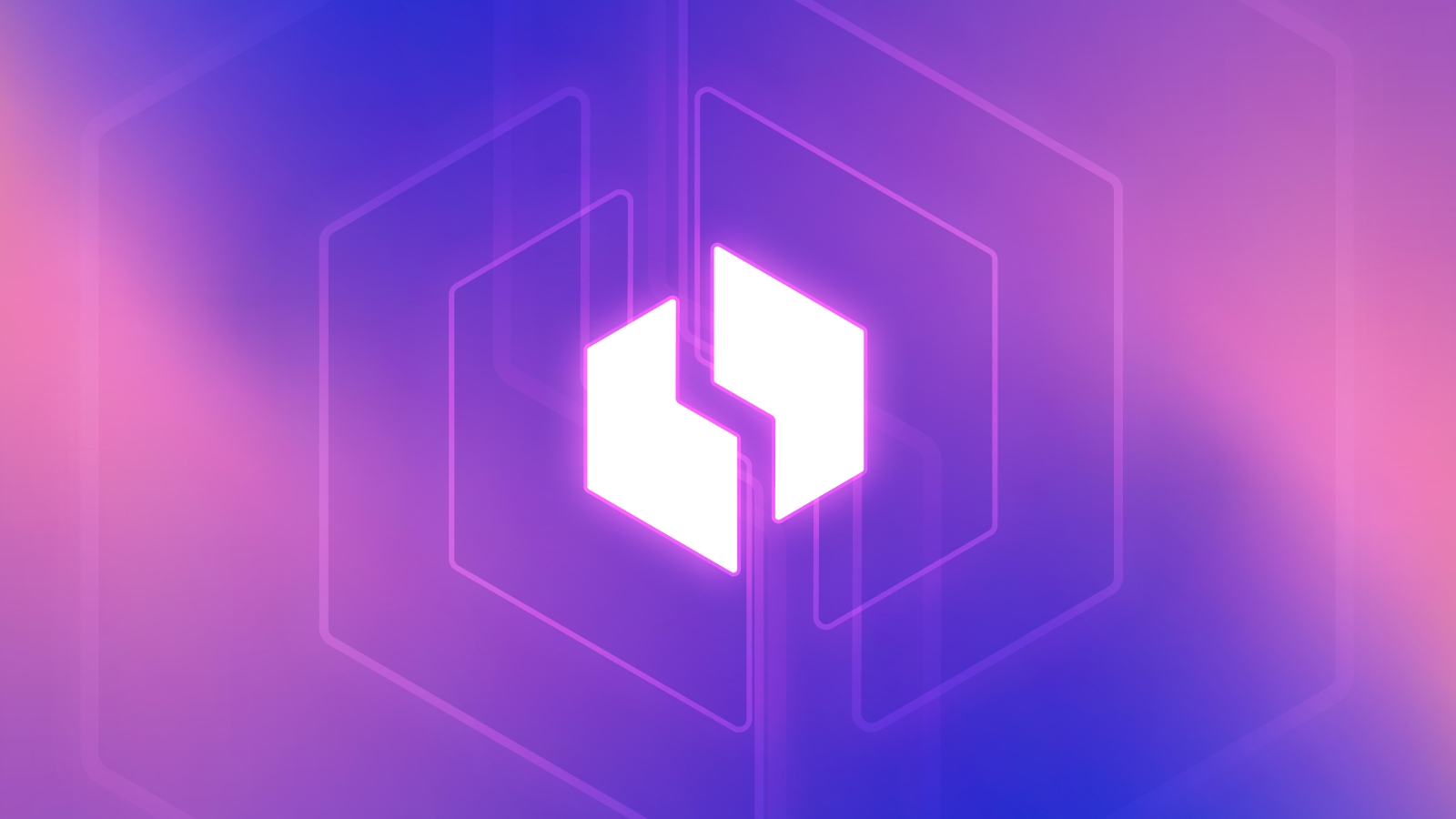
20시간 전
Enhancing Context Recall in Retrieval-Augmented GenerationRetrieval-augmented generation (RAG) has emerged as a pivotal method for integrating large language models (LLMs) into specialized business applications, enabling the infusion of proprietary data into model responses. Despite its effectiveness during the proof of concept (POC) phase, developers often face significant accuracy drops when transitioning RAG into production. This issue is particularly pronounced during the retrieval phase, where the aim is to accurately fetch the most relevant context for a given query, a metric known as context recall. This article delves into strategies for enhancing context recall by customizing and fine-tuning embedding models, ultimately improving RAG's performance in real-world applications.
RAG operates in two main steps: retrieval and generation. In the retrieval phase, the model converts text into vectors, indexes, retrieves, and re-ranks these vectors to identify the top matches. However, failures in this phase can lead to missed relevant contexts, resulting in lower context recall and less accurate generation outputs. One effective solution is to adapt the embedding model, which is designed to understand relationships between text data, to produce embeddings that are specific to the dataset being used. This fine-tuning allows the model to generate similar vectors for similar sentences, enhancing its ability to retrieve context that is highly relevant to the query.
To improve context recall, it is essential to prepare a tailored dataset that reflects the types of queries the model will encounter. This involves extracting a diverse range of questions from the knowledge base, paraphrasing them for variability, and organizing them by relevance. Additionally, constructing an evaluation dataset helps assess the model's performance in a realistic setting. By employing an Information Retrieval Evaluator, developers can measure metrics like Recall@k and Precision@k to gauge retrieval accuracy. Ultimately, fine-tuning the embedding model can lead to substantial improvements in context recall, ensuring that RAG remains accurate and reliable in production environments.